Manuscript accepted on :06-02-2023
Published online on: 24-10-2023
Plagiarism Check: Yes
Reviewed by: Dr. Rajendran Susai
Second Review by: Dr. Liudmila Spirina
Final Approval by: Dr. Anton R Kiselev


Vivek Kumar1, Kapil Joshi1*, Rajesh Kumar2, Harishchander Anandaram3
, Vivek Kumar Bhagat4
, Dev Baloni5
, Amrendra Tripathi6
, Minakshi Memoria1
1Department of Computer Science, Uttaranchal Institute of Technology, Uttaranchal University, Dehradun, India
2Department of Computer Science, Meerut Institute of Technology, Meerut, India
3Centre for Computational Engineering and Networking, Amrita School of Engineering, Coimbatore, Amrita Vishwa Vidyapeetham, India
4Amity University, Uttar Pradesh , Noida, India
5Quantum School of Technology, Quantum University, Roorkee, India
6School of Computer Science, UPES, Dehradun, India
Corresponding Author Email:kapilengg0509@gmail.com
DOI : https://dx.doi.org/10.13005/bpj/2772
Abstract
Multimodal medical image fusion is the efficient integration of various imaging modalities to improve the ability to assess, direct therapy, treat patients, or predict outcomes. As image fusion offers additional essential information, the correctness of the image generated from different medical imaging modalities has a substantial impact on the success of a disease's diagnosis. A single medical imaging modality cannot provide complete and precise information. In the modern research area, multimodality medical image fusion approach is one of the meaningful research in the area of medical imaging and radiation medicine. The fusion of medical images is the process of enrolling and combining multiple images from one or more imaging modalities, enhancing the image quality and to achieve randomness and redundancy, heighten the clinical utility of medical images in the diagnosis and evaluation of medical problems. The thought is to enhance the image occurrence Magnetic resonance imaging (MRI) is achieved by combining images like computerized tomography (CT) as well as magnetic resonance imaging (MRI) gives fine soft tissue information as long as CT gives fine facts over denser tissue. In this research paper, we have an account that features for future development with demanding performance requirements and processing speed.
Keywords
Discrete Wavelet Transform; Image Fusion; Multimodality; Quality Measurement Technique; Spatial Domain; Transform Domain
Download this article as:
Copy the following to cite this article: Kumar V, Joshi K, Kumar R, Anandaram H, Bhagat V. K, Baloni D, Tripathi A, Memoria M. Multi Modalities Medical Image Fusion Using Deep Learning and Metaverse Technology: Healthcare 4.0 A Futuristic Approach. Biomed Pharmacol J 2023;16(4). |
Copy the following to cite this URL: Kumar V, Joshi K, Kumar R, Anandaram H, Bhagat V. K, Baloni D, Tripathi A, Memoria M. Multi Modalities Medical Image Fusion Using Deep Learning and Metaverse Technology: Healthcare 4.0 A Futuristic Approach. Biomed Pharmacol J 2023;16(4). Available from: https://bit.ly/46KnoI0 |
Introduction
Uncommonly, images have independent or defined content of information. Images of various sorts reflect various forms of information. It produces the information that is dispersed and impairs the doctor’s judgment. This issue has drawn the interest of an abundance of experts in the area of medical diagnosis. Image fusion is an efficient technique which can be done automatically determine the data contained in unlike images as well as incorporate them to yield one composite image containing all of the objects at stake that are simple and clear 1. Yet, the overall performance of diagnosis is determined by the visual quality but also by information contained in medical images 2.Presently, there are various imaging modalities attainable to acquire particular medical information of a specific organ by human brain X-ray, positron emission tomography, MRI, and CT, as well as computed tomography based on single-photon emission (SPECT) images revealed in Figure 1 are vital medical imaging techniques through them. The image fusion mechanism is capable of efficiently taking action on this challenge. Enriching the data provided by merging the additional information given by combining two may be more modalities to create a single image 3.
![]() |
Figure 1: Classification of different multi-model imaging |
The medical images collected from sensors from various sources are fused in order to enlarge the diagnostic image modality’s quality 4.
From 2016 to 2021, the technology of medical image fusion has advanced significantly, as can be seen in Figure 2.
![]() |
Figure 2: Number of Articles published to Image Fusion 5 |
The goal of this study is just to outline the advancement of exploration and the future advancements of this entire field, bringing it together with regard to scientific publications around fusion of medical images in modern years. This article is divided into sections listed below 6:
An overview of contemporary fusion methods
Discrete Wavelet Transform using Haar
The Proposed Fusion Method
Quality Measurement Technique to test the MSE, PSNR, and SSIM methods to determine their compatibility.
Fusion Methods
Depending on the spatial domain, this same fusion procedure is based on the transform domain and the deep learning fusion method 7.
Spatial Domain
Spatial Domain Fusion technology is being developed is not difficult, because the fusion methods can be done directly enforced to the pixels of the original image, forcing the combined image. We directly accord with pixels and cast the pixels to obtain a fused resultant image 8. During pixel techniques, image fusion techniques frequently guide to the nonexistence of spectral information and propose spatial alterations. It consists of many methods, such as the Mean Method and the Select Maximum Procedure, Choose the Minimalist Approach, Intensity Hue Saturation Fusion Method, etc 9. These methods of fusion occurs directly enforced in relation here to input images and that will reduce the final fused image’s signal-to-noise ratio by the straight forward averaging method 10.
Average Method
The Average method is a fusion approach used to join images by averaging the pixels. This approach is intent on all parts of the image and if the images are captured same from the same form of sensor then it works correctly. Assuming that the images have high brightness and high contrast, then it will give a good outcome 11.
It’s described as:

Where, F(x, y) is the final fused image, P(x, y) and Q(x, y) are two input images
Select Maximum Method
In it, the result of the fused image is attained by choosing the maximum corresponding pixel intensity in contrast to the pair of input images.

In this case, A and B were also input images, and F is the fused image 11.
Select Minimum Method
In it, the result of the fused image is attained by choosing the minimum corresponding pixel intensity in contrast to the pair of input images.

In this case, A and B were also input images, and F is the fused image 11.
Intensity Hue Saturation (HIS) Fusion Method
The three attributes of a specific color that create a regulated visual representation of an image are intensity, hue, and saturation. The oldest technique of image fusing is the HIS transform. Hue and Saturation contain most of the spectral information and must be carefully controlled in HIS space 12. It has certain characteristics: (1) the intensity additive has nothing to do with the image’s information on color. (2) Saturation and hue additives are carefully associated with the manner human beings understand color 13. Hence, this technique is used to figure out the color issue in the image fusion steps.
![]() |
Figure 3: Steps of HIS Fusion |
Transform Domain
Transformation Domain Medical image fusion method is primarily according to the theory of multi-scale transformation (MST). The transformation domain-based method of fusing medical images translates original image taken from the time domain to obtain a low frequency domain or any other domains and high-frequency coefficients 14.
The most frequently used transform domain fusion techniques for medical images:
Discrete Fourier Transform
Discrete Cosine Transform
Discrete Wavelet Transform
Discrete Fourier Transform
It is crucial that image processing software is used to break down an image transformed into cosine and sine elements. The result of the transform represents a Fourier transform as well as a frequency range image, but the input image is the same as an image in real space. In the image of the Fourier domain, a particular point shows a specific frequency composed of the image of the spatial domain. It is utilized in broad applications such as image reconstruction, image filtering, and image analysis image processing and image compression are all examples of processing images 15. In the case of an oblong representation of size N * N, in two dimensions, DFT is described as:

This can be an equation explained as the worth of points F (k, l) is captured, such as adding to a spatial picture appropriate primary performance and adding the results.
The basic objective with cosine and sine waves rising frequencies, i.e. F(0,0) means the image’s DC element correlates in comparison to the typical brightness, as well as F(N-1, N-1) reflects the frequency at its peak.
At the same, the Fourier representation could be changed back into the area of space. Fourier’s inverse convert is defined as:

Take note of 1/N2. The reverse conversion includes a normalization term. To achieve a result from the above equation, a double sum must be calculated from a particular point of view. However, because the Fourier Transform seems to be separable, it could be used separately and described as:

Applying both of these equations, an image in the spatial domain is represented first by converting and using N Fourier transforms in one dimension, create an image in the middle. After that, the middle image is converted from the finished image, repeatedly adopting there are N Fourier Transform in one dimension. Compelling the 2-D regarding a Fourier Transform sequence of 2N 1-dimensional transforms reduces the quantity of needed approximation 16.
Transforming Fourier outcomes is an output image with a complex numerical value that can be shown because of two images, either genuine or made up of parts or size and polarity. Only the size of an image processing employs the Fourier Transform process, usually as it is displayed, consists of the majority of the data on the geometrical design of the image in the spatial domain.
Discrete Cosine Transform
DCT is a 4-step transformation coding method. The source image is first divided into sub-blocks measuring 8 * 8 pixels 17. Then, each block is converted to a frequency domain representation using a 2-dimensional DCT basis function. The following frequency coefficients are quantized and lastly, lossless entropy encoder output. DCT is an efficient image compression method because it can de-correlate image pixels.
The equation for 1-DCT

Where, k = 0,1 ………… N-1
The equation for 2-DCT:

Here is Figure 4, which provides the basic idea of DCT.
![]() |
Figure 4: Basic idea of DCT |
Discrete Wavelet Transform
It is a time domain analysis approach with a fixed window size and convertible forms. In the high frequency section of discrete wavelet transform converted signals, the time differentiated rate is excellent. In addition, the frequency difference rate in the low frequency section is good. It is capable of successfully extracting information from a signal 18.
Figure 5 depicts a two-channel, one-dimensional filter bank for perfect reconstruction. Filters for low and high analysis are utilized to convolve the input discrete sequence x. Each of the two down sampled signals, xH and sL is transformed according to the algorithm. For well-created filters, x is a signal precisely reconstructed (y=x) 19.
![]() |
Figure 5: A two-chennel perfect reconsruction filter bank |
In a 2-Dimensional Discrete Wavelet Transform, a 1-Dimensional DWT is performed in rows first, then by using filters but also down sampling data columns separately. These outcomes are in three types of detail coefficients and one specify of approximation coefficient describing the diagonal, vertical, and horizontal, planes of the image. To theory of filter terminology, four of these secondary images are equivalent toward the results of the low-low (LL), low-high (LH), high-low (HL), high-high (HH) bands. Fig.6 represents an image decomposition on a single level f(x, y) into four sub-bands LL, LH, HL, HH. Hence, a DWT with N-levels of degradation will have M = 30N + 1 similar spectrum bands. Figure 6 shows the 2-Dimensional Wavelet Transform structures with a single level of decomposition.
![]() |
Figure 6: 2-D DWT multi-resolution images decomposition 20 |
The statement for the 2-D DWT in the image is given by

Where m and n parameters relocate and a is a scaling factor Ψ(m, n) transform using the Haar wavelet.
Figure7 represents the components using a two-channel two-filter bank for perfect dimensional reconstruction.
![]() |
Figure 7: DWT structure with labeled sub bands |
DWT Using Haar
This is the merged image with multiple scales for each level of the image, merging the correlations to get a highest, lowest, or average blending path for every image 21. The concluding next would be the image reconstruction of images to obtain the concluding merged image by applying IDWT to the output value multiplied by the level of obtaining the output as depicted in figure8.
![]() |
Figure 8: Fusion process of DWT using Haar |
Deep Learning
In recent years, the study of image fusion has expanded to include deep learning. One popular deep learning model is the convolutional neural network. The activity level measurement (feature extraction) and fusion rules deficiencies in spatial and transform domain-based image fusion approaches necessitate artificial design, and the association between them is negligibly minor. Convolutional neural network is a trainable supervised learning multistage feed forward artificial neural network. Convolution is a multidimensional operation. In a convolutional network, the primary parameter is typically known as an input, and the second parameter is known as a kernel function, and the output is known as a function map. Sparse representations, parameter sharing, and isomorphic representations are the three main architectural ideas of convolutional neural networks 22. However, convolutional networks and types of sparse representation of neurons connect only to a few neighboring neurons in the previous stage and perform local convolution operations, thus reducing memory requirements and increasing computational efficiency. The CNN stage has constant weights and better memory requirements than other stages.
Convolutional Neural Network
Since medical images have different intensities at the same points, the fusion approach proposed isn’t always appropriate for scientific picture fusion. This approach makes use of the Siamese network to create a weight map. Since the two weight branches are the same, the function extraction or pastime stage size strategies of the supply image are the same23. It has advantages over pseudo-Siamese and two-channel models, and the ease of training Siamese models is also the reason why they are preferred for fusion applications. After getting the weight maps, Gaussian pyramid rework is used and pyramid transform is used for multi-scale decomposition, so the merging process is more consistent with human visual perception. Furthermore, we adaptively adjust the decay coefficients using a localized similarity-based fusion strategy. This combines the pyramid and similarity-based fusion algorithms with the CNN model to produce a superior fusion method. Figure 9 is an example algorithm.
![]() |
Figure 9: Schematic diagram besed on CNN fusion algorithm |
Metaverse
“Metaverse” is a term that refers to the digital world that exists outside the real world. It can be viewed as a 3D virtual world that allows users to interact and engage with each other. It is based on block chain technology and uses crypto currencies known as exchange traded commodities (ETPs) to pay for goods and services. The metaverse is still in active development. A three-tier architecture is shown in fig. 10. A fundamental requirement of the metaverse is that the architecture must move from the physical world to the virtual one 24,25.
![]() |
Figure 10: Three-layer Architecture of the Metaverse |
Some of the key promises of the metaverse are:
Decentralized world: Metaverse is a decentralized world where users can manage their own data.
Identity verification: Metaverse uses block chain technology to verify user identities and ensure that only authorized users can access data.
Smart contracts: The metaverse uses smart contracts to automate transactions.
ETPs: Metaverse native crypto currencies can be used to pay for goods and services, or reward AI applications for task completion.
The Proposed Model
The suggested approach is a multimodal utilized fusion specifically for images in medicine. This method seeks to achieve more clinically relevant use of medical imaging for the evaluation and evaluation of health issues by reducing redundant work, and improving quality.
An issue needs to be resolved first by starting images with how to process and obtain the best image match from the suggested method. This is because the images that were taken by the patient are divided into various shapes and sizes. Choosing and finding the ideal match between images is really challenging for the naked eye, so prior to applying any proposed technique, we need to order that match. The subsequent algorithm flowchart is presented as shown in figure 11.
Flow chart for the Proposed Image Fusion Method
![]() |
Figure 11: The flowchart steps for the proposed image fusion |
The process starts by analyzing the image utilizing data from two different sources finding various sizes. If the operation does not connect, the action stops, but if the size matches, the activity continues. Then, by identifying the difference in error between each tested image, the variation between the two images is determined, and the best following match between the two images is determined. They apply DWT as a future step to splitting the two images into four coefficients at a predetermined level and approach every level coefficient. Applying yields the final fused images, the Inverse Discrete Wavelet Transform (IDWT) as an interpretation of the fusion of the image.
Proposed Image Fusion Algorithm
The suggested procedure a definition of an algorithm in stages like this:
Step 1: Read the images from CT and find the size.
Step 2: Read the images from the MRI and find the size.
Step 3: Find the best matching images.
Step 4: Apply discrete wavelet transform.
Step 5: Apply the fusion selection rule to the images.
Step 6: Take the inverse discrete wavelet transform.
Step 7: Get a fused image of the output.
Quality Measurement Technique
In this research paper, we have to test the MSE, PSNR and SSIM methods to determine their compatibility. There are various ways to improve image quality widely used to access and access peak signal over noise ratio, universal image quality index, and mean square error, among others, Human Vision System, Features Similarity Index Technique, Structured Similarity Index Technique etc 26.
Mean Square Error (MSE)
It represents the squared mean difference between the original image and the filtered image. The roots you can estimate mean square error in one of several methods to measure the disparity between the values of an estimate as well as the actual certificated quality 27. Mean Square Error is provided by the following formula, in which M and N are dimensions (width and height) of the images, the improved image I (i, j), as well as the original image K (i, j). The row or column pixel of the basic and improved image is represented by the letters i and j 28.

If the MSE detects two identical images, it is zero. The PSNR for this value is not defined (zero division). An image the same as the original produces an unclear PSNR, given that the MSE becomes zero due to a division-by-zero error.
Peak Signal-To-Noise Ratio (PSNR)
The maximum signal-to-noise ratio is the ratio peak power of an indication of the loudness the signal’s strength. In order to gauge how well the motion estimation method performs in the suggested system, PSNR. As a metric, the peak based on the mean square error has been employed of quality and the following calculation can be used to determine its value 27:
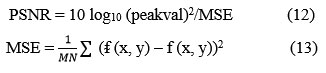
The frequency of pixels in an image is represented here by MN. ᵮ (x, y) the decompressed image is, and f (x, y) is the initial image.
Structure Similarity Index Measure ( SSIM)
SSIM is used to determine the degree of resemblance between two images. It is the measurement of image quality using an original reference or noise-free image as a baseline and a noisy image as a deformed image. In this, two images are compared based on luminous, contrast and structure [27]. The equation that is used to calculate the value of two images A and B is:

Where,
The mean values μA and μB are the functions of x, y, σ2A and σ2B are the variances, σAB is the covariance.
Results
Images include the presence of axial brain tumor (MRI) and CT. After receiving the fused image, we have to test the MSE, PSNR and SSIM. The results are described in Table 1.
Table 1: Results of all different fusion techniques
No. |
Fusion Techniques |
MSE |
PSNR |
SSIM |
1 |
Mean, Mean |
0.2132 |
1.223 |
1.10 |
2 |
Mean, Max |
0.2134 |
2.333 |
2.20 |
3 |
Mean, Min |
0.3332 |
1.273 |
1.14 |
4 |
Min, Min |
0.2232 |
2.423 |
1.21 |
5 |
Min, Max |
0.2434 |
1.223 |
1.88 |
6 |
Max, Min |
0.3132 |
2.423 |
1.08 |
7 |
Max, Max |
0.1932 |
2.413 |
2.33 |
8 |
Max, Mean |
0.2034 |
2.423 |
3.20 |
9 |
Min, Mean |
1.3232 |
3.223 |
2.13 |
Conclusion
In image processing, IQA is crucial. There are several sorts of image quality metrics in use for determining image quality. The optimum quality metric is obtained in this article by using MSE, PSNR, and SSIM. This paper combines images using a DWT and the average rule. The fused image quality is improved by testing and analyzing by using metrics. Different medical services, including illness prevention, healthcare, disease diagnosis and treatment, management of chronic diseases, consultation, etc., could be facilitated by the interaction between virtual and real cloud professionals and terminal users.
Conflict of Interest
There are no conflict of interest.
Funding Sources
There is no funding sources.
References
- Yi Li, Junli Zhao, Zhihan Lv, Jinhua Li. Medical Image Fusion Method By Deep Learning. International Journal Of Computer And Communication Engineering. 2021; 2: pp. 21-29. doi: https://doi.org/10.1016/j.ijcce.2020.12.004.
CrossRef - Nalini S. Jagtap, Sudeep D. Thepade. Application of Multi-Focused and Multimodal Image Fusion Using Guided Filter on Biomedical Images. International Conference on Big data Innovation for Sustainable Cognitive Computing. 2022: pp. 219-237. doi: https://doi.org/10.1007/978-3-031-07654-1_16.
CrossRef - Srinivasu Polinati, Durga Prasad Bavirisetti, Kandala N V P S Rajesh, Ganesh R Naik, Ravindra Dhuli. The Fusion of MRI an CT Medical Images Using Variational Mode Decomposition. Applied Science. 2021; 11(22): 10975. doi: https://doi.org/10.3390/app112210975.
CrossRef - Harpreet Kaur, Deepika Koundal, Virender Kadyan. Image Fusion Techniques: A Survey. Springer. 2021; 28: pp. 4425-4447. doi: https://doi.org/10.1007/s11831-021-09540-7.
CrossRef - Bing Huang, Feng Yang, Mengxiao Yin, Xiaoying Mo, Cheng Zhong. A Review Of Multimodal Medical Image Fusion Techniques. Hindawi Computational And Mathematical Methods In Medicine. 2020; 2020: 8279342. doi: https://doi.org/10.1155/2020/8279342.
CrossRef - Kangjjan He, Jian Gong, Dan Xu. Focus-pixel estimation and optimization for multi-focus image fusion. Multimedia Tools and Applications. 2022; 81: pp. 7711-7731. doi: https://doi.org/10.1007/s11042-022-12031-x.
CrossRef - Akram Alsubari, Ghanshyam D. Ramteke, Rakesh J. Ramteke. Transformation Of Voice Signals To Spatial Domain For Code Optimization In Digital Image Processing. Communications In Computer And Information Science. 2021; 1381: pp.196-209. doi: https://doi.org/10.1007/978-981-16-0493-5_18.
CrossRef - Bing Huang, Feng Yang, Mengxiao Yin, Xiaoying Mo, Cheng Zhong. A Review Of Multimodal Medical Image Fusion Techniques. Hindawi Computational And Mathematical Methods In Medicine. 2020; 2020: 8279342. doi: https://doi.org/10.1155/2020/8279342.
CrossRef - Harpreet Kaur, Deepika Koundal, Virender Kadyan. Image Fusion Techniques: A Survey. Springer. 2021; 28: pp. 4425-4447. doi: https://doi.org/10.1007/s11831-021-09540-7.
CrossRef - Tuba Kurban. Region based multi-spectral fusion method for remote sensing images using differential search algorithm and IHS transform. Experts Systems with Applications. 2022; 189: 116135. doi: https://doi.org/10.1016/j.eswa.2021.116135.
CrossRef - Padmavathi K, Maya V Karki. An Efficient PET-MRI Medical Image Fusion Based On HIS-NSCT-PCA Integrated Method. International Journal Of Engineering And Advanced Technology. 2019; 9(2) : pp.2073-2079. doi: https://doi.org/10.35940/ijeat.B3365.129219.
CrossRef - M.N.Do and M.Vetterli. The Contourlet Transform: An Efficient Directional Multiresolution Image Representation. IEEE Transactions On Image Processing. 2005; 14(12): pp. 2091-2106. doi: https://doi.org/10.1109/TIP.2005.859376.
CrossRef - Mohammed H. Rasheed, Omar M. Salih, Mohammed M. Siddeq, Marcos A. Rodrigues. Image Compression Based On 2D Discrete Fourier Transform And Matrix Minimization Algorithm. J. Array. 2020; 6: 100024. doi: https://doi.org/10.1016/j.array.2020.100024.
CrossRef - Amaefule I., Agbakwuru A O, Elei F O. Development Of Image Authentication Application Using Frequency Domain Digital Watermarking System. International Journal Of Advances In Engineering And Management. 2022; 4(4): pp. 1195-1199. doi: http://dx.doi.org/10.35629/5252-040411951199.
- Amina Belalia, Kamel Belloulata, Shiping Zhu. Efficient Histogram For Region Based Image Retrieval In The Discrete Cosine Transform Domain. IAES International Journal Of Artificial Intelligence. 2022; 11(2): pp. 546-563. doi: http://dx.doi.org/10.11591/ijai.v11.i2.pp546-563.
CrossRef - Javad Abbasi Aghamaleki, Alireza Ghorbani. Image Fusion Using Dual Tree Discrete Wavelet Transform And Weights Optimization. The Visual Computer. 2022. doi: https://doi.org/s00371-021-02396-9.
- Gundugonti Kishore Kumar, Mahammad Firose Shaik, Vikram Kulkarni and Rambabu Busi. Power and Delay Efficient Haar Wavelet Transform for Image Processing Application. Journal of circuits, systems and computers. 2022; 31(8). doi: http://dx.doi.org/10.1142/S0218126622200018.
CrossRef - Risheng Liu, Zhu Liu, Jinyuan Liu, Xin Fan. Searching a Hierarchically Aggregated Fusion Architecture for Fast Multi-Modality Image Fusion. ACM International Conference on Multimedia. 2021: pp. 1600-1608. doi: https://doi.org/10.1145/3474085.3475299.
CrossRef - N. Tawfik, H.A. Elnemr, M. Fakhr, M.I. Dessouky, Abd El-Samie. Survey study of multimodality medical image fusion methods. Multimedia Tools Applications. 2021; 80: pp. 6369–6396. doi: https://doi.org/10.1007/s11042-020-08834-5.
CrossRef - Haihan Duan, Jiaye Li, Sizheng Fan, Zhonghao Lin, Xiao Wu, Wei Cai. Metaverse for Social Good: A University Campus Prototype. ACM International Conference on Multimedia. 2021: pp. 153-161. doi: https://doi.org/10.1145/3474085.3479238.
CrossRef - Hassan Ahmed El Shenbary, Ebeid Ali Ebeid, Dumitru Baleanu. COVID-19 classification using hybrid deep learning and standard feature extraction techniques. Indonesian Journal of Electrical Engineering and Computer Science. 2023; 29(3): pp. 1780-1791. doi: http://dx.doi.org/10.11591/ijeecs.v29.i3.pp1780-1791.
CrossRef - Umme Sara, Morium Akter, Mohammad Shorif Uddin. Image Quality Assessment Through FSIM, SSIM, MSE and PSNR – A Comparative Study. Journal Of Computer And Communications. 2019; 7(3): pp. 8-18. doi: https://doi.org/10.4236/jcc.2019.73002.
CrossRef - Suhel Kaur, Sumeet Kaur. Fractal Image Compression – A Review. International Journal of Advanced Research. 2016; 4(7): pp. 322-326. doi: http://dx.doi.org/10.21474/IJAR01.
CrossRef - Piorkowski, R., Mantiuk, R. Calibration Of Structural Similarity Index Metric To Detect Artifacts In Game Engines. Computer Vision And Graphics. 2016; 9972: pp. 86-94. doi: https://doi.org/10.1007/978-3-319-46418-3_8.
CrossRef - Diwakar, M., Tripathi, A., Joshi, K., Memoria, M., Singh, P. Latest trends on heart disease prediction using machine learning and image fusion. Materials Today: Proceedings. 2021; 37(2): pp. 3213-3218. doi: https://doi.org/10.1016/j.matpr.2020.09.078.
CrossRef - Joshi, K., Diwakar, M., Joshi, N. K., & Lamba, S. A Concise Review on Latest Methods of Image Fusion. Recent Advances in Computer Science and Communications. 2021; 14(7): pp. 2046-2056. doi: http://dx.doi.org/10.2174/2213275912666200214113414.
CrossRef - Joshi, K., Joshi, K. N., Diwakar, M., Tripathi, N. A., & Gupta, H. Multi-focus image fusion using non-local mean filtering and stationary wavelet transform. International Journal of Innovative Technology and Exploring. Engineering. 2019; 9(1): pp. 344-350. doi: https://doi.org/10.35940/ijitee.A4123.119119.
CrossRef