Manuscript accepted on :08-10-2024
Published online on: 24-10-2024
Plagiarism Check: Yes
Reviewed by: Dr. Elina Marinho
Second Review by: Dr. Bhuvana R
Final Approval by: Dr. Prabhishek Singh


Govindamoorthi Paramasivam, Ranjith Kumar Paulraj
and Vimala Mannarsamy*
Department of ECE, P.S.R Engineering College, Sivakasi, Tamilnadu, India.
Corresponding Author E-mail: vimala@psr.edu.in
DOI : https://dx.doi.org/10.13005/bpj/3039
Abstract
The problem of Atherosclerosis diagnosis and prediction have been well studied and there are numerous classifier Algorithms were designed by various Researchers to predict and classification of atherosclerosis diseases. However, these algorithms suffer to achieve higher performance in predicting and diagnosing the disease according to the samples given. To address this issue, the research has developed an efficient Real-Time Invariant Atherosclerosis Feature Selection and Classification Model (RIFSACM). This method focused on choosing optimal features and improves the performance of classifications. It fetches given dataset and applies an Invariant Feature Normalization Technique (IFNT) to remove the noisy features or tuples and also eliminates noisy records from the dataset. Moreover, an Invariant Multi Feature Nominal Clustering (IMFNC) method groups the tuples of dataset under various class of Atherosclerosis. During the testing phase, an Invariant Atherosclerosis Multi-Feature Dependent Classifier (IAMFDC) algorithm is introduced to classify test samples into various categories of atherosclerosis. This classifier algorithm estimates the value of Multi Factor Disease Dependent Weight (MFDDW) against various classes of diseases to perform classification. The proposed method enhances both classification and feature selection performance, achieving accuracy, sensitivity, and specificity rates of 98.2%, 98.36%, and 100%, respectively.
Keywords
Atherosclerosis; Classification; Disease Prediction; Feature Selection; IAMFDC; IMFNC; MFDDW
Download this article as:
Copy the following to cite this article: Paramasivam G, Paulraj R. K, Mannarsamy V. Efficient Disease Prediction with a Real-Time Invariant Atherosclerosis Classification and Feature Selection Model. Biomed Pharmacol J 2024;17(4). |
Copy the following to cite this URL: Paramasivam G, Paulraj R. K, Mannarsamy V. Efficient Disease Prediction with a Real-Time Invariant Atherosclerosis Classification and Feature Selection Model. Biomed Pharmacol J 2024;17(4). Available from: https://bit.ly/40ihrBU |
Introduction
The human society has great influence from various diseases which affect them for long or short term damages. When the diseases are affecting in temporary way, then it does not make any permanent idleness in the human life. Some of the diseases are identified as more harmful which makes permanent damage to the life of human 1. Coronary Artery Disease (CAD) is a serious global health problem caused by plaque buildup in the coronary arteries and is the leading cause of death worldwide. According to the World Health Organization (WHO), millions of people die from CAD each year, and it is predicted that by 2040, more than 30 million people could die from heart failure. Atherosclerosis, the condition responsible for CAD, involves the accumulation of plaque that narrows the artery lumens, restricting Oxygen-rich blood flow to the heart. This reduced blood flow can lead to insufficient oxygen for the heart muscles, causing pain in the arms, neck, shoulders, and chest, known as angina. A blockage of the blood supply can result in a heart attack. Obesity, high blood cholesterol, asthma, overweight, high blood pressure, and other risk factors can increase the risk of heart and vascular disease2. Alcohol and stress are two other factors that may rise the risk of complications from heart failure. Therefore, there is a need to develop and implement medical diagnostic support systems (MDSS) to automate the diagnosis and prognosis of cardiovascular diseases in patients. Additionally, more accurate and efficient medical diagnosis research is required for making wise clinical judgements. Although the conventional approaches MDSS have demonstrated their capacity to handle the majority of diagnostic issues. When the person affected by CAD, there will be different issues arises in the part of the body and accordingly, there will be malfunction in the human organs. For example, when the wall of artery gets deposited with cholesterol it becomes more rigid and it loses the property of fluctuation which in turn produces heart attacks, strokes and so on. When the blood supply being stopped to the brain it produces stroke where the choke in the blood supply to the heart leads to heart attack. The prompt and accurate diagnosis of cardiac failure is challenging due to various obstacles. Therefore, developing a cost-effective CAD intelligence model to predict cardiac disease at an early stage is crucial. Early detection and understanding of symptoms can significantly reduce CAD. Researchers have found that several factors increase the likelihood of heart failure. The presence of disease can be identified with several features and it supports the curation of the disease when it is diagnosed at the earliest. Disease prediction and classification are the process of identifying the possible disease according to the set of symptoms given to the algorithm. There exist numerous classifiers like K-means, Swarm Whale, LeNet and so on. Each classifier works on the set of features considered and they would estimate different similarity measures for the given sample and separate various disease classes. Based on the similarity measure only, they perform classification. But the performance is varying greatly according to the measurement of similarity and the set of features considered. Here, the feature selection becomes most important and the performance of classification is greatly depending on the set of features being used and how the similarity among the samples is measured. There are numerous classifier Algorithms were designed by various Researchers to predict and classification of atherosclerosis diseases. However, these algorithms suffer to achieve higher performance in predicting and diagnosing the disease according to the samples given. To address this issue, the research has introduced an efficient Real-Time invarient Atherosclerosis Feature Selection and Classification Model (RIFSACM). This method focused on choosing optimal features and improves the performance of classifications. It fetches given dataset and applies an Invariant Feature Normalization Technique (IFNT) to remove the noisy features or tuples and also eliminates noisy records from the dataset. Moreover, an Invariant Multi Feature Nominal Clustering (IMFNC) method groups the tuples of dataset under various class of Atherosclerosis. At the testing stage, an Invariant Atherosclerosis Multi Feature Dependent Classifier (IAMFDC) algorithm is introduced to classify the test sample against different class of atherosclerosis. This classifier algorithm estimates the value of Multi Factor Disease Dependent Weight (MFDDW) against various classes of diseases to perform classification. The detailed approach is discussed in this paper.
The rest of the paper is structured as follows: Section 2 provides the background of the study. Section 3 addresses the Time Invariant Feature Selection and Atherosclerosis Classification Model. Section 4 presents the simulation results and discussion. Finally, Section 5 provides the conclusion and outlines the future scope of the work.
Related Works
The problem of atherosclerosis disease detection and prediction were discussed by various researchers. Innovative techniques for predicting atherosclerosis risk factors, including imputation algorithms and particle swarm optimization (PSO) 3, has been proposed. This method identifies physical inactivity as a key factor in assessing risk. Various heterogeneous data models4 have been proposed to analyze the progression of atherosclerosis (ATS) in coronary vessels. A detailed review of machine learning algorithms5 in predicting the coronary artery disease were considered different approaches and compare its performance with other state-of-art techniques. Machine learning based high risk predicting model 6 was designed to find the location of the plaque and performs classification with support vector machine. A medical decision support system (MDSS) of atherosclerosis disease 7 was proposed to use K means clustering, K-Nearest Neighborhood classification and Artificial Neural Network8. Machine learning model with combination of statistical analysis were used to reduce information redundancy and improve the accuracy of disease diagnosis9. A bio sensor assisted deep learning 10 model and U-Net++ ensemble algorithm 11 were discussed to identify the plaques from the images and perform classification. Similarly, a tissue characterization method was proposed to train with Convolutional Neural Network (CNN) model of arterial pathology towards classification 12. A mathematical and stochastic model were studied towards monitoring the plaque vulnerability by detecting the inflammatory. The method was capable of detecting as well as finding the progress of the plaque 13.
Six widely used advanced technologies have been introduced to measure physiological parameters: imaging photoplethysmography (IPPG), laser Doppler, radio frequency (RF), thermal imaging (TI), fiber optic sensing, and piezoelectric sensors. These techniques use video imaging to capture subtle changes in skin color that correspond to heart-synchronized fluctuations in blood volume in subcutaneous arteries and capillaries. The laser Doppler technique assesses blood flow by analyzing the spectral components of light reflected from illuminated tissue surfaces. RF technology relies on Doppler shifts caused by the periodic movement of the chest wall due to respiration and heartbeat. TI measures vital signs by detecting electromagnetic radiation emitted from blood flow. Optical fiber sensors detect changes in light properties resulting from interactions between the physiological parameter being measured and the incoming light. Piezoelectric sensors utilize the piezoelectric effect of dielectric materials. 14. A deep-learning convolutional neural network was developed towards classifying the atherosclerosis where the model is constructed with the plaque features extracted from the images 15. A multitask recurrent convolutional neural network was designed to extract features and supports towards classification 16. A novel wearable ultrasonic imaging assembly was proposed for routine monitoring of the carotid arteries in an easy-to-use and economical way 17. A deep learning-based study of intravascular ultrasound atherosclerotic plaque development was analyzed that extracts the features from plaque images and applies PCA-net towards classification 18. A machine learning based predicting coronary artery disease according to the plaque location 19, identification of risk factors of atherosclerosis20 and wall shear stress based machine learning model21 were proposed that uses the relations among various parameters like blood density, velocity, viscosity and so on. Further a multivariate linear regression, multilayer perceptron neural network and Gaussian conditional random fields (GCRF) were presented. Deep neural network models, including convolutional neural networks (CNN) and recurrent neural networks (RNN)22 used to group features extracted by bidirectional layers.
Class imbalance is a significant issue often encountered in machine learning applications. Consensus clustering based under-sampling approach was adopted for imbalanced learning. In the consensus clustering methods, five clustering algorithms—k-means, k-modes, k-means++, self-organizing maps, and the Divisive Analysis (DIANA) algorithm—along with their combinations, were considered23. Intelligent machine learning technique, multiclass SVM was used for assorting the individuals24. Feature selection is crucial for developing robust and efficient classification models, as it helps reduce training time. Individual filter-based feature selection methods are commonly used due to their simplicity and relatively high performance25. Deep learning, a recent advancement in machine learning, aims to improve classification performance through multiple layers of nonlinear information processing. The extrapolative performance of various supervised machine learning methods, such as Naïve Bayes, support vector machines (SVM), logistic regression, k-nearest neighbors, and random forests, as well as three ensemble learning methods, has been evaluated26. Latent Dirichlet Allocation (LDA) addresses the high dimensionality issue of vector space models, but its effectiveness depends on identifying the right parameter values. A swarm-optimized approach is used to estimate LDA parameters, including the number of topics and other relevant parameters. Additionally, a hybrid ensemble pruning method, which combines diversity actions and clustering, is designed to create a multiple classifier system with enhanced predictive performance and greater diversity27 All the above discussed approaches suffer to achieve higher performance in classifying atherosclerosis in higher accuracy. This work aims to propose a novel Real-Time Invariant Feature Selection and Atherosclerosis Classification Model (RIFSACM) for diagnosing patients with atherosclerosis-related heart diseases. The study utilizes four machine learning algorithms: Invariant Feature Normalization Technique (IFNT), Invariant Class-Specific Target Support Feature Selection (ICSTS), Invariant Multi-Feature Nominal Clustering (IMFNC), and Invariant Atherosclerosis Multi-Feature Dependent Classifier (IAMFDC). The performance of these algorithms in classifying the atherosclerosis model is then evaluated.
Materials and Methods
A Novel Real-Time Invariant Feature Selection and Classification Framework
The proposed real-time invariant feature selection and atherosclerosis classification model (RIFSACM) are started by reading the given dataset. From the dataset, the method generates the preprocessed dataset by applying Invariant Feature Normalization Technique (IFNT) which removes the noisy features or tuples and eliminates noisy records from the dataset. Further, the tuples of the dataset has been grouped under various disease classes according to the application of Invariant Multi Feature Nominal Clustering (IMFNC) scheme. Third, the method classifies the data samples by applying Invariant Atherosclerosis Multi Feature Dependent Classifier (IAMFDC) which estimates the value of Multi Factor Disease Dependent Weight (MFDDW) against various classes of diseases to perform classification. The design of the RIFSACM model is depicted in Figure 1, which involves in various operations towards the classification of atherosclerosis and the various parts are detailed in this section
![]() |
Figure 1: Architecture of Proposed RIFSACM Model |
Invariant Feature Normalization Technique (IFNT)
The atherosclerosis dataset includes various features related to individuals, such as Age, Sex, Weight, Height, Body Mass Index (BMI), Cholesterol, Smoking, Drinking, Lipid Profile, and other diagnostic results. However, the data set has been collected from various sources which contains heterogeneous form and needs to be normalized. In order to use the data set towards the classification of atherosclerosis for any given sample, the data set should be normalized. This method initially finds the set of features which are common in most tuples of the dataset. Once the maximum features are identified, then tracks the presence of all the features in any data point. If the data point covers the required features, then it has been kept with the dataset, otherwise it has been removed from the set. On the other side, towards normalization, the method estimates the Invariant Tuple Normalization Value (ITNV) for various features. The value of ITNV is measured according to the mean value of the feature and frequency of tuples higher than mean value. Depending on the value of ITNV, the approach normalizes the missing feature values and restores them to support effective disease prediction and classification. The normalized dataset has been used to perform classification on atherosclerosis.
The features in the dataset have been identified as follows:

// Where Ads is the Atherosclerosis Data set and Fls is the feature list.
Towards normalization, the method estimates value mean for all the features as follows:

Further the method computes the frequency of samples with the feature value higher than the mean as follows:

The above discussed algorithm performs preprocessing and normalization on the given atherosclerosis dataset and identifies the noisy records and also, remove them from the set. Further, the method normalizes the feature values to support disease prediction and classification.
Invariant Class Specific Target Support Feature Selection
The proposed feature selection algorithm finds a subset of features from the available list based on their importance in various classes of tuples. It has been performed by computing invariant class specific target support (ICSTS) value for different features. Based on the value of ICSTS, the approach finds a subset of attributes from the list. It has been measured based on the occurrence of the feature in specific class of disease tuple and other disease class tuples. By computing their frequency values in various classes, it estimates the value of ICSTS to perform feature selection. Selected features are used towards clustering the dataset under various groups as well as to perform classification.
The value of ICSTS is measured by computing frequency support (FS) ,Value support (VS), Preprocessed Dataset (Prd) and Random value (Rd). The value of FS is measured as follows:
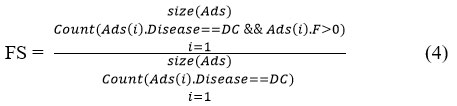
//where Ads is the Atherosclerosis Data set and DC is the disease class.
Similarly, the value of value support (VS) is measured as follows:
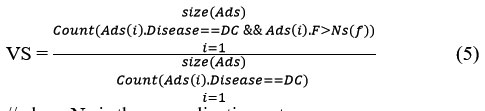
//where Ns is the normalization set.
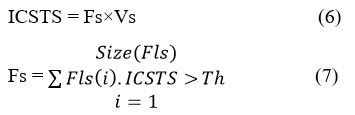
The above discussed algorithm performs feature selection based on the value of ICSTS measured for various features. Based on the subset of features selected, the method performs clustering as well as classification.
Invariant Multi Feature Nominal Clustering (IMFNC).
The proposed approach groups the disease data sets under different class of atherosclerosis using invariant multi feature nominal clustering algorithm. The clustering algorithm assigns random samples to the different clusters and with the remaining samples, the method computes Invariant Atherosclerosis Support (IAS) towards various class of diseases according to the features of the sample given. According to the value of IAS, the approach assigns the sample to the specific class which is being iterated for number of times. Generated clusters are used towards classification at the test phase.
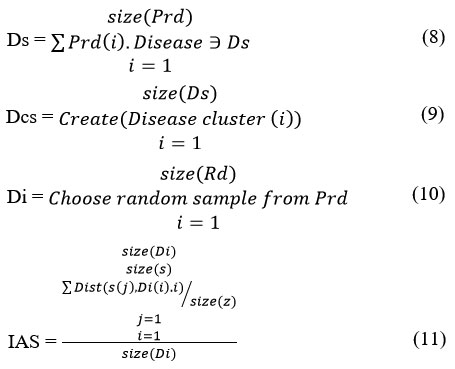
The above discussed algorithm estimates IAS value for the samples of the data set and iterates the same till there is no swap identified between any clusters. The generated cluster has been used for classification in a later stage.
IAMFDC Classification
The proposed approach performs classification of atherosclerosis disease according to the invariant features present in the sample given. The method computes Multi Factor Disease Dependent Weight (MFDDW) against various classes of diseases to perform classification. To measure the value of MFDDW, the method computes Lipid Profile support (LPS), Physical profile support (PPS) and Lifestyle support (LS). Using all these support values, the method computes the MFDDW value, which is then used for classification.
The classification process computes the Lipid Profile support (LPS) as follows:

//where Di is the disease class, Ch-Cholestrol, Pr-Pressure, Th-threshold value.
Similarly, the method computes physical profile support (PPS) as follows

//where w-is the weight, BMI-Body mass index and Th is threshold.
Finally, the value of lifestyle support is measured as follows:
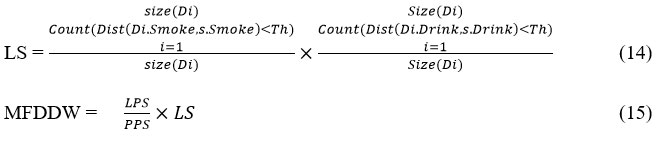
The above discussed algorithm performs classification of data sample by computing MFDDW value for the sample according to various support measures. Based on this value, the method conducts the classification.\
Results and Discussion
The proposed invariant multi feature atherosclerosis classification algorithm has been implemented and evaluated their performance under various circumstances. The method has been evaluated for its performance by using the Kaggle dataset obtained from the open source platform. It is known that insufficient training data can lead to inaccurate approximations. An over-constrained model may underfit a small dataset, while an under-constrained model is likely to overfit the data, both of which result in poor performance. Similarly, having too little test data can lead to an overly optimistic and highly variable estimate of the model’s performance. The results produced by the approach have been compared with the results of other approaches. Table 1 presents the details of the dataset used to evaluate the performance of the proposed model. Accordingly, the performance of the method is measured under various metrics and presented in this section.
Table 1: Evaluation Details
Factor |
Value |
Data Set |
Kaggle (Cardio Vascular Disease) |
Number of Features |
12 |
Number of Tuples |
50000 |
Number of Classes |
2 |
The dataset consists of 50000 records of patient’s data in 12 features, such as age, gender, systolic blood pressure, diastolic blood pressure, and etc. The target class “cardio” equals to 1, when patient has cardiovascular disease, and its 0, if patient is healthy. The task is to predict the presence or absence of cardiovascular disease (CVD) using the patient assessment results. There are 3 types of input features:
Objective: factual information.
Examination: results of medical examination.
Subjective: information given by the patient.
Features:
Age | Objective Feature | age | int (days)
Height | Objective Feature | height | int (cm) |
Weight | Objective Feature | weight | float (kg) |
Gender | Objective Feature | gender | categorical code |
Systolic blood pressure | Examination Feature | ap_hi | int |
Diastolic blood pressure | Examination Feature | ap_lo | int |
Cholesterol | Examination Feature | cholesterol | 1: normal, 2: above normal, 3: well above normal |
Glucose | Examination Feature | gluc | 1: normal, 2: above normal, 3: well above normal |
Smoking | Subjective Feature | smoke | binary |
Alcohol intake | Subjective Feature | alco | binary |
Physical activity | Subjective Feature | active | binary |
Presence or absence of cardiovascular disease | Target Variable | cardio | binary |
Table 2: Analysis on Atherosclerosis Classification Performance
Analysis on Atherosclerosis Classification Performance % |
|||
Methods |
15000 Samples |
30000 Samples |
50000 Samples |
PSO |
69 |
74 |
79 |
UNet Ensemble |
73 |
77 |
83 |
GCRF |
77 |
82 |
87 |
RIFSACM |
86 |
92 |
97 |
Table 2 The performance of the methods in classification is shown to vary with different numbers of samples in the dataset. In each case, the proposed method improves the performance than other approaches. The ratio of false classifications produced by various approaches is measured and presented in Table 3 and RIFSACM produced less false ratio compare to other approaches. The proposed method was compared with existing optimization approaches, and the results indicate that the new system outperforms traditional methods. The proposed approach achieved a higher classification performance rate of 86%, compared to the existing PSO method, which had a lower accuracy rate of 69% with 15,000 samples. Additionally, the existing PSO approach exhibited a higher false positive rate of 31%, while the proposed method achieved a lower false positive rate of approximately 14%.
Table 3: Analysis on False Classification Ratio
False Ratio in Atherosclerosis Classification % |
|||
Methods |
15000 Samples |
30000 Samples |
50000 Samples |
PSO |
31 |
26 |
21 |
UNet Ensemble |
27 |
23 |
17 |
GCRF |
23 |
18 |
13 |
RIFSACM |
14 |
8 |
3 |
![]() |
Figure 2: Analysis on Atherosclerosis Classification Performance |
The classification performance produced by various approaches are measured and compared in Figure 2, where the proposed RIFSACM has produced higher classification accuracy than other approaches.
![]() |
Figure 3: False classification Ratio |
The ratio of false classification introduced by various approaches are measured and compared in Figure 3, which denotes, the proposed RIFSACM has produced less false ratio than other approaches.
Accuracy
It defines ordered errors to calculate the arithmetic field of the composition. Low accuracy results in a discrepancy between measurements and actual values. This approach ensures that exceptional data samples are repeatedly evaluated by the same algorithm, leading to accurate testing by the computer or device. The precision of the final results is represented as the percentage of correct outcomes.

Sensitivity
Sensitivity, often referred to as the true positive rate, measures the proportion of actual positives correctly identified. It is calculated as the percentage of true positive cases accurately detected by the model.

Specificity
Specificity, also known as the true negative rate, measures the proportion of actual negatives that are correctly identified. It calculates the percentage of true negative cases accurately detected by the model.

Where TP= True Positive, FP- False Positive, TN- True Negative, FN- False Negative
Table 4: Analysis on various performance metrics
Methods |
Accuracy |
Sensitivity |
Specificity |
PSO |
87.85 |
97 |
92 |
UNet Ensemble |
91.42 |
98.6 |
77 |
GCRF |
95.97 |
100 |
93.7 |
RIFSACM |
98.2 |
98.36 |
99.7 |
The performance metrics on Accuracy, Sensitivity and Specificity are evaluated and compared in Table 4, where the proposed RIFSACM approach has produced higher performance in all the metrics.
![]() |
Figure 4: Analysis on sensitivity |
The ratio of sensitivity produced on the classification has been measured and compared with the results of RIFSACM approach in Figure 4. However, the proposed RIFSACM demonstrates higher sensitivity compared to other methods. Figure 4 shows the sensitivity rates for different approaches, with the proposed system achieving a sensitivity of 98.36%, while the existing PSO, UNet Ensemble, and GCRF methods show sensitivities of 97%, 98.6%, and 100%, respectively. Figure 6 illustrates the comparative performance analysis in terms of accuracy. The proposed method outperforms existing techniques with an accuracy rate of 98.2%, whereas the PSO method has the lowest accuracy. This indicates that the proposed method is more effective overall. Additionally, performance evaluations reveal that the proposed method also has better specificity compared to other approaches.
![]() |
Figure 5: Analysis on specificity |
The ratio of specificity achieved in the classification has been measured and compared with the results of the RIFSACM approach, as shown in Figure 5. However, the proposed RIFSACM demonstrates higher specificity compared to other approaches. The accuracy of the classification has been assessed and compared with that of other methods, as illustrated in Figure 6. The proposed RIFSACM also achieves superior accuracy relative to the other approaches.
![]() |
Figure 6: Analysis on accuracy |
Conclusion
This paper presented a real-time invariant feature selection and atherosclerosis classification model (RIFSACM). The method first applies Invariant Feature Normalization Technique (IFNT) which removes the noisy features or tuples and eliminates noisy records from the dataset. Second, the Invariant Multi Feature Nominal Clustering (IMFNC) scheme was adapted to perform clustering and the method classifies the samples using Invariant Atherosclerosis Multi Feature Dependent Classifier (IAMFDC) which estimates the value of Multi Factor Disease Dependent Weight (MFDDW) against various classes of diseases to perform classification. The experimental results showed that the RIFSACM has produced a better performance compared to other machine learning methods (PSO, U-Net Ensemble and GCRF) Techniques. The performance was assessed using various metrics including accuracy, specificity, sensitivity, recall, F-measure, precision, false negative rate (FNR), false positive rate (FPR), true negative rate (TNR), true positive rate (TPR), negative predictive value (NPV), and positive predictive value (PPV) for the proposed optimization, datasets, and classifier. According to the typical performance metrics indicator, this comparison portrayed that our proposed system has 98.2% as the highest accuracy, sensitivity as 98.36 % and specificity as 100 % in predicting and classification of atherosclerosis disease on the test dataset. The proposed approach improves the performance in clustering as well as classification with higher accuracy. In the future, the effectiveness of the proposed algorithm will be evaluated using a range of anatomical atherosclerosis medical imaging techniques.
Acknowledgement
We would like to thank P.S.R Engineering College for providing the resources that made this research possible.
Funding Sources
The author(s) received no financial support for the research, authorship, and/or publication of this article.
Conflicts of Interest
The author(s) do not have any conflict of interest
Data Availability Statement
This statement does not apply to this article.
Ethics Statement
This research did not involve human participants, animal subjects, or any material that requires ethical approval.
Informed Consent Statement
This study did not involve human participants, and therefore, informed consent was not required.
Clinical Trial Registration
This research does not involve any clinical trials
Author Contribution
Ranjith Kumar Paulraj and Vimala Mannarsamy: Contributed to the design of the Atherosclerosis Classification and Feature Selection Model.
Govindamoorthi Paramasivam: Responsible for the conceptualization of the manuscript. All authors have reviewed and endorsed the content of this work.
References
- Al-Ssulami A. M, Alsorori R. S, Azmi A. M, and Aboalsamh H. Improving Coronary Heart Disease Prediction Through Machine Learning and an Innovative Data Augmentation Technique. Cognitive Computation, 2023:1-16.
CrossRef - Dhanka S, Bhardwaj V. K, and Maini S. Comprehensive analysis of supervised algorithms for coronary artery heart disease detection. Expert Systems. 2023:e13300.
CrossRef - Rao V. S. H. and Kumar M. N. Novel Approaches for Predicting Risk Factors of Atherosclerosis. IEEE Journal of Biomedical and Health Informatics. 2013; 17(1): 183-189.
CrossRef - Exarchos KP, Carpegianni C, Rigas G, Exarchos TP, Vozzi F, Sakellarios A, Marraccini P, Naka K, Michalis L, Parodi O and Fotiadis DI. A Multi-scale Approach for Modeling Atherosclerosis Progression. IEEE Journal of Biomedical and Health Informatics. 2015; 19 (2):709-719.
CrossRef - Khidirova C, Sadikova S, Mukhsinov S, Nashvandova G and Mirzaeva S. Machine learning methods as a tool for diagnostic and prognostic research in cardiovascular disease. International Conference on Information Science and Communications Technologies (ICISCT). 2021:1-6.
CrossRef - Zhang L, Wahle A, Chen.Z, Lopez J.J, Kovarnik T and Sonka M. Predicting Locations of High-Risk Plaques in Coronary Arteries in Patients Receiving Statin Therapy. IEEE Transactions on Medical Imaging. 2018; 37(1): 151-161.
CrossRef - Terrada O, Cherradi B, Raihani A and Bouattane O. Classification and Prediction of atherosclerosis diseases using machine learning algorithms. 5th International Conference on Optimization and Applications (ICOA). 2019: 1-5.
CrossRef - Oumaima Terrad and Bouchaib Cherradi. A novel medical diagnosis support system for predicting patients with atherosclerosis diseases. Elsevier Informatics in Medicine Unlocked, 2021; 21: 100483.
CrossRef - Zihan Chen and Minhui Yang. Prediction of atherosclerosis using machine learning based on operations research. AIMS Press. Mathematical Biosciences and Engineering, 2022; 19(5): 4892-4910.
CrossRef - Hongliang Yang and Zinan Li. Prediction of atherosclerosis diseases using biosensor-assisted deep learning artificial neuron model Periodicals. Neural Computing and Applications. 2021; 33(10): 5257–5266.
CrossRef - Zhou R, Guo F, Azarpazhooh MR, Hashemi S, Cheng X, Spence JD, Ding M and Fenster A. Deep Learning-Based Measurement of Total Plaque Area in B-Mode Ultrasound Images. IEEE Journal of Biomedical and Health Informatics. 2021; 25(8): 2967-2977.
CrossRef - Olender M.L, Athanasiou L.S, Michalis L.K, D, Fotiadis D.I and Edelman E.R. A Domain Enriched Deep Learning Approach to Classify Atherosclerosis Using Intravascular Ultrasound Imaging. IEEE Journal of Selected Topics in Signal Processing. 2020; 14(6): 1210-1220.
CrossRef - Al-Zubi M M, and Mohan. A. S. Implantable Biosensor Interface Platform for Monitoring of Atherosclerosis. IEEE Sensors Letters. 2020; 4(2): 1-4.
CrossRef - Luo J, Yan Z, Guo S and Chen W. Recent Advances in Atherosclerotic Disease Screening Using Pervasive Healthcare. IEEE Reviews in Biomedical Engineering, 2022; 15:293-308.
CrossRef - Li YC, Shen TY, Chen CC, Chang WT, Lee PY and Huang CJ. Automatic Detection of Atherosclerotic Plaque and Calcification from Intravascular Ultrasound Images by Using Deep Convolutional Neural Networks. IEEE Transactions on Ultrasonics Ferroelectrics, and Frequency Control. 2021. 68(5): 1762-1772.
CrossRef - Zreik M, Van Hamersvelt R. W, Wolterink J. M, Leiner T, Viergever M. A and Išgum I. A Recurrent CNN for Automatic Detection and Classification of Coronary Artery Plaque and Stenosis in Coronary CT Angiography. IEEE Transactions on Medical Imaging, 2018; 38(7): 1588-1598.
CrossRef - Shomaji S, Dehghanzadeh P, Roman A, Forte D, Bhunia S and Mandal S. Early Detection of Cardiovascular Diseases Using Wearable Ultrasound Device. IEEE Consumer Electronics Magazine, 2019; 8(6): 12-21.
CrossRef - Zhan J, Wang J, Ben Z, Ruan H and Chen S. Recognition of Angiographic Atherosclerotic Plaque Development Based on Deep Learning. IEEE Access. 2019; 7: 170807-170819.
CrossRef - Zhang L, Wahlev A, Chen Z, Lopez J. J, Kovarnik T, and Sonka M. Predicting Locations of High-Risk Plaques in Coronary Arteries in Patients Receiving Statin Therapy, IEEE Transactions on Medical Imaging. 2018; 37(1): 151-161.
CrossRef - Al-Absi H. R. H, Refaee M. A, Rehman A. U, Islam M. T, Belhaouari S. B, and T. Alam. Risk Factors and Comorbidities Associated to Cardiovascular Disease in Qatar: A Machine Learning Based Case-Control Study, IEEE Access. 2021; 9: 29929-29941.
CrossRef - Jordanski M, Radovic M, Milosevic Z, Filipovic N and Obradovic Z. Machine Learning Approach for Predicting Wall Shear Distribution for Abdominal Aortic Aneurysm and Carotid Bifurcation Models. IEEE Journal of Biomedical and Health Informatics. 2022; 22(2): 537-544.
CrossRef - Aytug Onan. Bidirectional convolutional recurrent neural network architecture with group-wise enhancement mechanism for text sentiment classification. Journal of King Saud University- Computer and Information Sciences. 2022; 33(5): 2098-2117.
CrossRef - Aytug Onan. Consensus Clustering-Based Undersampling Approach to Imbalanced Learning. Hindawi Scientific Programming. 2019, 3: 1-14.
CrossRef - Kumar P.R and Priya M. Intelligent approaches for prognosticating atherosclerotic and non-atherosclerotic individuals. 2014
International Conference on Communication and Signal Processing. 2014: 691-695.
CrossRef - Aytug Onan. A feature selection model based on genetic rank aggregation for text sentiment classification, Journal of Information Science. 2016; 43(1).
CrossRef - Aytug Onan. Mining opinions from instructor evaluation reviews: A deep learning approach, Computer Applications in Engineering Education. 2019;28(1): 117-138.
CrossRef - Aytug Onan. Biomedical text categorization based on ensemble pruning and optimized topic modelling. Computational and Mathematical Methods in Medicine. 2018: 1-22.
CrossRef