Manuscript accepted on :15-02-2024
Published online on: 08-03-2024
Plagiarism Check: Yes
Reviewed by: Dr. Dito Anurogo and Dr. Jothiraj S
Second Review by: Dr. Sharath B.S
Final Approval by: Dr. Najam A Siddiqi


Asaad Ma. Babker1*, Rania Saad Suliman2
, Rabab Hassan Elshaikh 3
, Sardor Boboyorov4
and Vyacheslav Lyashenko5
1Department of Medical Laboratory Sciences, College of Health Sciences, Gulf Medical University, Ajman, UAE
2Department of Clinical Laboratory Sciences, Prince Sultan Military College for Health Sciences, Dhahran, Saudi Arabia
3Department of Medical Laboratory Sciences , A'Sharqiyah University, Ibra,Oman
4Tashkent Medical Academy Termiz branch, Termiz, Uzbekistan
5Department of Media Systems and Technology, Kharkiv National University of Radio Electronics, Kharkiv, Ukraine
Corresponding Author E-mail:azad.88@hotmail.com
DOI : https://dx.doi.org/10.13005/bpj/2842
Abstract
Platelets play an important role in the diagnosis and detection of various diseases, the course of the disease in the future, and the possibility of justifying treatment methods. In this aspect, platelet counting is of key importance. For these purposes, it is important to correctly identify such objects. This served as the basis for the development and generalization of an appropriate medical image analysis procedure. The purpose of this study is to construct a generalized procedure for platelet identification in medical digital images. The work examined at least 30 images containing objects such as platelets. These images are approximately the same type, but with different intensity of the presence of the main objects in the blood smear. Similar but noisy images are also considered. These images were noisy with different types of noise. Thus, a total of at least 120 images were examined. In general, this allows us to evaluate the effectiveness of the proposed procedure for identifying platelets in medical images. This procedure includes simple methods of image analysis such as: binarization, morphological analysis, taking into account the influence of the sizes of different objects and comparative analysis of images at intermediate stages of the study. To summarize the results, estimates such as the percentage of false detection of platelets and the percentage of missed platelets were considered. The platelet identification results that were obtained for non-noisy images are as follows: false platelet isolation was less than 0.1%, missed platelets were within 2-2.5%. The worst result for noisy images is false platelet isolation – within 10% (for images with multiplicative noise), missed platelets – within 7.5-8% (for images with multiplicative noise). It should also be noted that noisy images are characterized by identification of platelets with distortion of their sizes: these sizes are reduced or increased. The percentage of such distortions does not exceed 1.3% (for images with Poisson noise).The problematic aspects of platelet identification in digital medical images are considered. Particular attention is paid to simple methods of digital image processing. Among the problematic aspects of the proposed approach there is a need to clearly take into account the geometric dimensions of platelets. The results obtained are acceptable and can be used as the basis for an automated blood smear analysis system.
Keywords
Digital Imaging; Identification; Medical Imaging; Platelets
Download this article as:
Copy the following to cite this article: Babker A. M, Suliman R. S, Elshaikh R. H, Boboyorov S, Lyashenko V. Sequence of Simple Digital Technologies for Detection of Platelets in Medical Images. Biomed Pharmacol J 2024;17(1). |
Copy the following to cite this URL: Babker A. M, Suliman R. S, Elshaikh R. H, Boboyorov S, Lyashenko V. Sequence of Simple Digital Technologies for Detection of Platelets in Medical Images. Biomed Pharmacol J 2024;17(1). Available from: https://bit.ly/3IzywNf |
Introduction
Analysis, as a component of the diagnostic process, plays an important role. Moreover, such an analysis should be considered not only as an element of diagnosis, but also as an element of research 1-3. This is most relevant for the medical field, when it is necessary to make the most effective decisions without dramatically affecting the human body. For these purposes, it is advisable to consider digital medical images. These images allow us to explore the possible problems of the issue based on non-contact analysis, considering the microcosm of a person.
Blood is primarily considered as the object of such research. Blood, as a liquid and mobile connective tissue of the internal environment of the body, allows one to judge the possibility of the development of many diseases 4-6 . Blood consists of a liquid medium – plasma, and formed elements suspended in it: erythrocytes, leukocytes and platelets7. There are many different works that study blood components such as red blood cells and white blood cells based on medical digital images 8-10. This is due to the relative simplicity of such analysis compared to medical imaging of blood elements such as platelets. In particular, this feature is the size of platelets.
Platelets also called thrombocytes are the smallest blood component produced from the very large bone marrow cells called megakaryocytes under controlling of thrombopoietin hormone and they play a fundamental role in thrombosis and hemostasis 11. Platelets, which circulate within the blood, are the essential mediators that trigger the mechanical pathway of the coagulation cascade upon encountering any damage to the blood vessels. Platelets encourage primary hemostasis via three major processes: activation, adhesion, and aggregation. When the integrity of the vascular endothelium is interrupted, various macromolecular elements of the vascular subendothelium become exposed and readily accessible to platelets 12,13. Platelets possess important secretory functions. During the process of activation, platelets express internal membrane proteins and release adhesive proteins, coagulation and growth factors. Some of the proteins facilitate the crosstalk of platelets with leukocytes and endothelial cells 14. The platelets function depends on the number (quantity) and structure (quality) and when platelets do not function properly, people are at risk of excessive bleeding due to injuries or even spontaneous bleeding 15. The normal platelet count in humans ranges from 150×109/L to 400×109/L. We take into account that platelets have a circulating lifespan of around 10 days, and that about one third of platelets are sequestered in the spleen. A constant balance is, therefore, required between thrombopoiesis, and platelet consumption and senescence 16. Low platelet concentration is called thrombocytopenia and is due to either decreased production or increased destruction. Elevated platelet concentration is called thrombocytosis, and is either congenital, reactive (to cytokines), or due to unregulated production 17.
Therefore, platelet counting is an important task in blood analysis and diagnosis of possible disease 18.
Now platelets are counting is included in a complete blood count (CBC), a panel of tests often performed as part of a general health exam by using hematology analyzer, this technique is based on the modification of the impedance of calibrated aperture soaking in an electrolyte and going through a constant course delivered by two electrodes located on both sides of the aperture then count sample are counted automatically 18. Up to date, the only “Gold Standard” in platelet counting available to assess any degree of accuracy of the automated count has been the manual phase-contrast microscopic method. The manual method itself has significant limitations in terms of performance, particularly in the area of imprecision 19.
Based on the discussion above, the main purpose of this study is to review some procedure for platelet identification in medical images. In the future, this procedure will be the basis for automatic platelet counting. Therefore, the basics of platelet identification using image processing technologies are discussed next, the procedure is summarized, and the results of the related analysis are presented.
Material and Methods
In order to identify platelets in medical images, it is advisable to consider some approaches to medical image processing. It should be emphasized that in this case it is necessary to pay attention to the so-called small-sized objects. This is based on the fact that the image of platelets refers to such objects.
A general technique for identifying objects in medical images is the use of binarization of the original image. This is because the blood smear is usually stained for phase contrast microscopy. In this case, components of the blood smear such as red blood cells, leukocytes and platelets differ significantly from the general background (plasma). Then it becomes possible to binarize the image and isolate the main components of blood. An example of the use of this approach is the study of M. Habibzadeh, A. Krzyzak, T. Fevens and A. Sadr 20 or B. Azam, R. J. Qureshi, Z. Jan and T. A. Khattak 21. But in the simplest case, it is advisable to use conventional binarization by threshold 22-24.
However, for example, S. Rahman, B. Azam, S. U. Khan, M. Awais and I. Al note that for effective binarization it is necessary to normalize the intensity of the input image 25. However, even in this case it is impossible to obtain an ideal resulting image. This is because many medical imaging devices experience non-linear brightness effects. Moreover, it can be enhanced as a result of uneven distribution of the coloring matter. Thus, some interference occurs which introduces errors in the corresponding identification. This problem can be solved by filtering the original image or processed image. But then some data may be lost, as noted by various authors 26, 27.
The complexity of solving the problem of binarization when isolating platelets in medical images is noted in 28. For these purposes, the authors use the droplet detection method, which considers the quantitative assessment of clinically significant signs of such droplets.
At the same time, the work 29 uses segmentation and machine learning procedures to solve binarization problems for medical images. For these purposes, the authors pre-process the original images, removing duplicate data and outliers. Ultimately, this simplifies platelet identification. However, in this case, the presence of a person is required to remove such unnecessary information.
In this case, to solve the problem, various morphological operations are used: combining individual elements, filling empty space, clarifying the boundaries of objects, etc. These operations are also widely used in processing various medical images and more 30, 31. In this context, we operate with objects that are predominantly round or close to a circle, which makes it easier to use such operations for our research.
The geometric dimensions of platelets should also be taken into account. This will help separate them from the white blood cells and red blood cells. In general, the geometry of an object plays an important role in the study of medical images 32. It is also advisable to compare the obtained images at different stages of the study. This method can be viewed as a logical analysis of the relationship between individual processing steps.
Then, among the main stages of platelet identification one can highlight: binarization, morphological analysis, taking into account the influence of the sizes of different objects and comparative analysis of images at intermediate stages of the study.
Thus, a generalized algorithm for identifying platelets on digital images can be presented in accordance with Fig. 1.
![]() |
Figure 1: Generalized algorithm for platelet identification in digital medical images |
The general mathematical formalization of the generalized algorithm for identifying platelets on digital images may be represented in next way:

where
Iv – original image,
Ib – image after the binarization procedure,
Ims – image after morphological processing taking into account platelet sizes. This is an image where there are only platelets,
Ik – comparative analysis, which allows platelets to be identified in the original image,
Ir – the resulting image after all stages of processing.
To implement the binarization procedure, a threshold (a) is selected, which allows you to select only those points that belong to platelet images. This can be done based on a histogram of where the platelet images are the brightest. Then if such a threshold is reached, the point on the input image is designated 1, otherwise – 0:

where
(i, j) – the current point of the image that is being examined.
Morphological analysis is performed based on standard image analysis techniques 21, 22, 27.
At the same time, the image comparison procedure consists of comparing the original image and the image after morphological processing, taking into account the platelet size. Then the procedure for obtaining the resulting image can be expressed as follows:
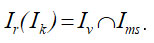
It is also important to emphasize that generalized result issues are also discussed here. This result was obtained based on the analysis of at least 30 different images where platelets are present. One of the typical such images is presented below. Thus, the platelet identification procedure for a certain class of medical images is actually considered. It should be emphasized that this work does not use any methods of statistical analysis and inference. The effectiveness of the proposed approach is determined by the sequence of implementation of known algorithms, which are themselves reliable. The performance of the procedure considered is also determined by the level of platelet identification and comparison of this result with known approaches (see the “Discussion” section). Additional research is also being carried out in the form of superimposing various noises (a few words about this below).
At the same time, the study noted the percentage of false detection of platelets and the percentage of missed platelets. In general, this gives a general picture of the performance of the considered procedure for identifying objects such as platelets.
To extend the related research, noisy images are also considered. To do this, various types of noise are superimposed on the original images. Moreover, this overlay of noise on an image is a standard procedure that is used in the development and analysis of various image processing procedures. In fact, this is an artificial distortion of real images. This allows one to understand the limits and reliability of some image analysis procedure, in this case platelet detection. However, such noise may not occur in real practice.
Results
Fig. 2 shows a typical image where platelets can be seen as round blue objects 33, 34. To the right is the original image, and to the left is part of it, as indicated by the corresponding arrow. These objects have different sizes and their colors also vary from light blue to dark blue.
![]() |
Figure 2: Example of original image with platelets. |
The figure below shows the results of binarization of the original image Fig. 2 (for the data in the picture to the right). This binarization is carried out based on the data of the corresponding histogram. This data can be unified and generalized to automatically binarize the original image. Here, the binarization threshold is selected in such a way that all elements of the blood smear except the background (plasma) are visible in the field of view.
![]() |
Figure 3: Results of binarization of the original image. |
The image after binarization has many errors in the form of empty areas in areas of interest, as well as many edge objects that may not be accurately identified. Therefore, morphological operations are used to eliminate such defects (see Fig. 4, cleaned binary image of blood smear). This identification step can also be automated.
![]() |
Figure 4: Original image after binarization and morphological operations |
Image in Fig. 4 includes all major blood smear objects except plasma. However, only platelets need to be isolated. This sequence is implemented in two stages. First, the platelet size data in the image was used (see Fig. 5a), and then compared Fig. 4 and Fig. 5a, only platelets are highlighted (see Fig. 5b). This procedure can be fully automated. At the same time, it is important to know the geometric dimensions of the platelets in the image.
![]() |
Figure 5: Isolation of platelets |
Platelets can now be identified in the original image. To do this, a logical comparison of the data is made Fig. 2 (for the data in the picture to the right) and Fig. 5b. The result is presented in Fig. 6.
![]() |
Figure 6: Platelet identification result for original image |
It should be noted that in this case, almost all platelets were identified with the exception of one, which has a non-standard geometric size. This confirms the fact that taking into account platelet size geometry is one of the key factors. However, no false platelets were detected in this case.
An analysis of the effectiveness of the considered procedure for identifying platelets in images that are subject to various types of noise was also carried out. The final results of such identification for this example are presented in Fig. 7. Here we consider such noise as: Gaussian white noise with zero mean value and variance 0.01; Poisson noise; multiplicative noise, with mean 0 and variance 0.05.
![]() |
Figure 7: Results of identifying platelets in an image that are subject |
Overall, the results are satisfactory. There is some degradation in the proposed platelet identification procedure. But this deterioration is not critical, as will be shown below. Moreover, the likelihood of exposure to such noise is unknown. This is artificial noise (see comments in the previous section). In this case, a violation of the geometric dimensions of platelets was also detected. But this violation is not significant (no more than 1.3% of such distortions from the total number of platelets). There was no statistical significance between size distortion and the number of false platelet detections or platelet identification in general. These results are presented in more detail in Tabl. 1 (summarized data for all images that were considered in the work).
Table 1: Platelet identification results based on the procedure reviewed
Image type |
Evaluations |
||
False platelet isolation, % |
Missed platelets, % |
Additionally |
|
Images without noise |
less than 0.1% |
within 2-2.5% |
– |
Images with Gaussian white noise |
within 1.5-2% |
no more than 6.2% |
Some areas of platelets are distorted: reduced or enlarged. No more than 1% |
Images with Poisson noise |
no more than 0.5% |
no more than 6.5% |
Some areas of platelets are distorted: reduced or enlarged. No more than 1.3% |
Images with multiplicative noise |
within 10% |
within 7.5-8% |
Some areas of platelets are distorted: reduced or enlarged. No more than 1.2% |
From the above data it is clear that the main problem is missed platelets. This problem is solved at the stage of image processing, taking into account the geometric dimensions of platelets. In particular, it is possible to isolate different groups of platelets, and then, after identifying them, combine everything into one. However, platelet size and its correct accounting is one of the factors that determines the scope of application of the proposed approach. Next we will also discuss other aspects of what was discussed above.
Discussion
As already noted, blood smear analysis and platelet identification in particular are the basis of many studies.
For example, work 35 considers the possibility of classifying platelets based on a combination of their statistical characteristics. This approach can be used as a basis when image analysis is performed taking into account the geometric dimensions of platelets. But here it should be taken into account that the accuracy of such classification, according to the authors, does not exceed 83.67% 35. Thus, this approach may introduce errors in platelet identification.
Study 36 describes a generalized automated procedure for counting platelets in a blood smear. For these purposes, platelet identification is based on the application of a segmentation procedure. However, such segmentation must take into account the color conditions of the blood smear, as well as the geometric dimensions of the platelets. Thus, the authors also face a number of problematic issues, which we also highlight. The authors offer their own solution to such issues, which in general does not contradict our approach.
The paper 37 considered the possibility of automating the counting of platelets in a blood smear to identify cases of dengue. This shows the importance of the problem we examined in our study. The authors justify simple solutions for such analysis. The work noted that the system they proposed in 11 cases out of 19 gave 100% results. But this result applies only to 59% of cases. In this regard, our result is more effective. At the same time, platelet skipping in other cases is 3-4%. However, the paper notes that automatic and manual platelet counting does not have statistical significance with a kappa coefficient of 0.6 35.
The study 38 focused on platelet detection and counting. This platelet identification is based on models such as Single Shot Multibox Detector (SSD), RetinaNet, Faster_rcnn and You Only Look Once_v3 (YOLO_v3). However, the authors pay attention to the YOLO_v3 model. As a result, more efficient platelet detection algorithms have been obtained. The modified YOLO_v3 model showed 1.8% higher average accuracy. The generalized efficiency of such identification is at the level of 86%. But there also remain issues of platelet false detection and missed detection.
The work 39 discusses platelet detection based on deep neural networks. The accuracy of this detection is about 86.7-91.2%, which is a pretty good result. In this case, an essential aspect of such an analysis is the possibility of overlapping individual elements of a blood smear. We did not consider such aspects in our study.
The works 28 and 29 addressed the issues of platelet detection in medical images. In 28 the authors use a droplet detection method based on clinically relevant features. This method allows for platelet detection at a level of 96.4%. The basis of such a calculation is a comparison with a similar calculation with the participation of an experienced laboratory assistant. The study 29 uses various classifiers, which provide an accuracy of identification of blood smear components at the level of 97-98%.
Y. K. A. A. Atmanto, A. A. Abdullah, D. Muhadi and M. Arif propose a method for determining the platelet count coefficient, which is analogous to the detection rate of such blood components 40. This ratio is the overall ratio divided by the sample size 40. The authors note that the total ratio of 254 samples was 4.086. This is a significant indicator. However, such data are difficult to compare with traditional platelet count summaries.
G. Drałus, D. Mazur and A. Czmil use the training method using deep learning networks RetinaNet 41 for platelet recognition purposes. The accuracy of platelet recognition is at the level of 92.5-97.36% (confidence threshold 0.35) 41. As the threshold increases, the accuracy of platelet identification decreases.
C. Briggs, P. Harrison, and S. J. Machin provide a comprehensive review of various platelet counting methods 42. In this case, special attention is paid to problematic aspects. In particular, the authors note that a decrease in the number of platelets in a blood smear leads to a decrease in the level of their identification. It is noted that the most inaccurate is the manual counting method, which leads to errors of various kinds at the level of 10-25%. It also talks about the influence of platelet size on the level of their detection in a blood smear. The main message of the study is that platelets are more difficult to count than red or white blood cells 42.
The study 43 examines the reference platelet count method. The authors note that this approach showed acceptable identification accuracy. No exact data is provided. However, it should be noted that this approach cannot be compared with our method. This is due to the fact that we do not use a reference image of platelets, but morphological and geometric features of platelets.
B. Mohamed-Rachid, A. F. Raya, A. H. Sulaiman and A. K. Salam compare and analyze different platelet counting methods 44. Among these methods are considered: optical, impedance, immunological and manual methods of platelet counting. The results showed that the reliability of similarity between such methods ranges from 0.49 to 0.9 44. The worst result is given by the impedance method, which overestimates the platelet count and at the same time does not give any result in 15% of samples (in other words, the overall platelet detection accuracy is below the 80% level). It is also noted that other authors note such a large error in the impedance method.
The work 45 discusses the process of selecting methods for automatic platelet counting. It is noted that the impedance detection method (PLT-I) can erroneously reduce platelet counts by as much as 23.6% 45. Then the level of platelet detection decreases to 76.4% or lower. However, it is also emphasized that there are few studies comparing the accuracy of platelet counts.
A generalized comparison of our platelet identification approach with several other methods is also presented below.
Table 2: Comparison of platelet identification results presented on a medical image.
Method |
Evaluations |
||
False platelet isolation, % |
Missed platelets, % |
Generalized identification accuracy, % |
|
Proposed approach |
less than 0.1% |
within 2-2.5% |
97.4% |
From [35] |
– |
– |
83.67% |
From [37] |
– |
3-4% |
59% |
From [38] |
– |
4-5% |
86% |
From [39] |
– |
– |
86.7-91.2% |
From [29] |
– |
– |
97-98% |
From [41] |
– |
– |
92.5-97.36% |
Manual counting method from [42] |
– |
– |
75-90% |
Impedance method from [44] |
– |
– |
under 80% |
Impedance method (PLT-I) from [45] |
– |
– |
76.4% or lower |
Tabl. 2 shows that the proposed approach is one of the top three methods for identifying platelets in medical images. Also noteworthy is the effectiveness of platelet detection under various interference conditions (see Tabl. 1). At the same time, the considered approach is distinguished by the simplicity of its implementation.
First of all, our approach when working with original images (where we do not artificially degrade such images with noise for research purposes) is at the level of developments that are presented in 29,41. Our result with such studies is at the level of 97.4% platelet identification.
In the case of various interferences, we obtain a platelet detection result of at least 82%. This result is comparable to the results from 35. The result obtained is also better than the manual counting method and impedance method (see Tabl. 2), which give less than 82% detection of platelets.
Thus, in general, it should be noted that our results are acceptable and allow us to create an automated system for identifying and counting platelets.
If we talk about the basics of such automation, we should note the possibility of its implementation based on the simplicity of the proposed approach. It should also be noted that our approach is clearly structured, which is based on the appropriate mathematical formalization. This allows us to apply different levels of automation. Such aspects will be considered in more detail in our further studies.
As for other problematic aspects in platelet identification, the following should be said. First of all, this concerns the problem of geometric dimensions of platelets. In this case, a preliminary procedure for analyzing platelet geometry and morphology can be introduced. Then, at the stage of applying platelet identification algorithms based on their geometry, it is possible to reduce the number of false results and increase the overall detection accuracy. The number of false results can also be reduced by creating a platelet bank for their subsequent identification with real data. You can also use algorithms based on the theory of fuzzy sets. This will speed up the platelet identification process and improve overall results. The use of fuzzy set theory approaches will facilitate the consideration of various types of images, the selection of the necessary steps to generate a general platelet detection procedure. To implement a friendly platelet detection procedure, it is proposed to use a potential communication interface in the implementation of such an analysis. This will allow us to understand and identify bottlenecks in the proposed approach and eliminate them.
Conclusion
The paper examines various problematic aspects of platelet identification in digital medical images. Particular attention is paid to simple methods of digital image processing. A sequence of actions has been proposed that allows for effective identification of platelets. The strength of the proposed approach is its simplicity and high efficiency in detecting platelets under various interferences that are possible in digital images. Among the problematic aspects of the proposed approach is the need to clearly take into account the geometric dimensions of platelets. A number of experiments were carried out on real images. The results obtained are acceptable and can be used as the basis for an automated blood smear analysis system.
At the same time, among the potential directions of this research and areas for improving the proposed procedure, we highlight:
Ddevelopment of a preliminary procedure for automatically determining platelet size in each series of corresponding input medical images;
Adaptation of the proposed approach to other types of input images.
Acknowledgement
None
Conflict of Interest
There is no conflict of interest.
Funding Source
There are no funding Sources.
References
- Rajula, H. S. R., Verlato, G., Manchia, M., Antonucci, N., & Fanos, V. (2020). Comparison of conventional statistical methods with machine learning in medicine: diagnosis, drug development, and treatment. Medicina, 56(9), 455.
CrossRef - Schubert, C. (2011). Making sure. A comparative micro-analysis of diagnostic instruments in medical practice. Social science & medicine, 73(6), 851-857.
CrossRef - Tsuneki, M. (2022). Deep learning models in medical image analysis. Journal of Oral Biosciences, 64(3), 312-320.
CrossRef - Aitekenov, S., Sultangaziyev, A., Abdirova, P., Yussupova, L., Gaipov, A., Utegulov, Z., & Bukasov, R. (2023). Raman, Infrared and Brillouin spectroscopies of biofluids for medical diagnostics and for detection of biomarkers. Critical Reviews in Analytical Chemistry, 53(7), 1561-1590.
CrossRef - Babker, A. M. (2020). The role of Inherited Blood Coagulation Disorders in Recurrent Miscarriage Syndrome. Journal of Critical Reviews, 7(1), 16-20.
- Rabotiahov, A., Kobylin, O., Dudar, Z., & Lyashenko, V. (2018, February). Bionic image segmentation of cytology samples method. In 2018 14th International Conference on Advanced Trends in Radioelecrtronics, Telecommunications and Computer Engineering (TCSET) (pp. 665-670). IEEE.
CrossRef - Reece, W. O. (2015). The composition and functions of blood. Reece, WO; Erickson, HH; Goff, JP; Uemura, EE Duckes’ Physiology of Domestic Animals 13th Edition, Wiley Blackwell, OX, UK, 114-136.
- Rahadi, I., Choodoung, M., & Choodoung, A. (2020, May). Red blood cells and white blood cells detection by image processing. In Journal of Physics: Conference Series, 1539(1), 012025.
CrossRef - Da Costa, L. (2015). Digital image analysis of blood cells. Clinics in laboratory medicine, 35(1), 105-122.
CrossRef - Lyashenko, V. V., Babker, A. M. A. A., & Kobylin, O. A. (2016). The methodology of wavelet analysis as a tool for cytology preparations image processing. Cukurova Medical Journal, 41(3), 453-463.
CrossRef - Periayah, Mercy Halleluyah et al. (2017). Mechanism Action of Platelets and Crucial Blood Coagulation Pathways in Hemostasis. International journal of hematology-oncology and stem cell research, 11(4), 319-327.
- Zhang, Y., Ehrlich, S. M., Zhu, C., & Du, X. (2022). Signaling mechanisms of the platelet glycoprotein Ib-IX complex. Platelets, 33(6), 823-832.
CrossRef - Rolling, C. C., & et al.. (2023). P2Y12 Inhibition Suppresses Proinflammatory Platelet–Monocyte Interactions. Thrombosis and Haemostasis, 123(02), 231-244.
CrossRef - Schrottmaier, W. C., Mussbacher, M., Salzmann, M., & Assinger, A. (2020). Platelet-leukocyte interplay during vascular disease. Atherosclerosis, 307, 109-120.
CrossRef - Haley, K. M. (2020). Platelet disorders. Pediatrics in review, 41(5), 224-235.
CrossRef - Daly, M. E. (2011). Determinants of platelet count in humans. Haematologica, 96(1), 10. 10-3.
CrossRef - Assinger, A. (2014). Platelets and infection–an emerging role of platelets in viral infection. Frontiers in immunology, 5, 649.
CrossRef - Mustafa, M. E., Mansoor, M. M., Mohammed, A., & Babker, A. A. (2015). Evaluation of platelets count and coagulation parameters among patients with liver disease. World Journal of Pharmaceutical Research, 4(10), 360-368.
- Bakhubaira S (2013) Automated Versus Manual Platelet Count in Aden. J Clin Exp Pathol 3: 149.
CrossRef - Habibzadeh, M., Krzyzak, A., Fevens, T., & Sadr, A. (2011, March). Counting of RBCs and WBCs in noisy normal blood smear microscopic images. In Medical Imaging 2011: Computer-Aided Diagnosis, 7963, 1009-1019.
CrossRef - Azam, B., Qureshi, R. J., Jan, Z., & Khattak, T. A. (2014). Color based segmentation of white blood cells in blood photomicrographs using image quantization. Res J Recent Sciences, 2277, 2502-9.
- Lyashenko, V., Kobylin, O., & Baranchykov, Y. (2018, October). Ideology of Image Processing in Infocommunication Systems. In 2018 International Scientific-Practical Conference Problems of Infocommunications. Science and Technology (PIC S&T) (pp. 47-50). IEEE.
CrossRef - Savanevych, V., Khlamov, S., Briukhovetskyi, O., Trunova, T., & Tabakova, I. (2023). Mathematical Methods for an Accurate Navigation of the Robotic Telescopes. Mathematics, 11(10), 2246.
CrossRef - Rajendran, S., & Kumar, E. S. (2019, July). Leukocytes Classification and Segmentation in Microscopic Blood Smear Image. In 2019 2nd International Conference on Intelligent Computing, Instrumentation and Control Technologies (ICICICT) (Vol. 1, pp. 1064-1068). IEEE.
CrossRef - Rahman, S., Azam, B., Khan, S. U., Awais, M., & Ali, I. (2021). Automatic identification of abnormal blood smear images using color and morphology variation of RBCS and central pallor. Computerized Medical Imaging and Graphics, 87, 101813.
CrossRef - Romero-Rondón, M. F., Sanabria-Rosas, L. M., Bautista-Rozo, L. X., & Mendoza-Castellanos, A. (2016). Algorithm for detection of overlapped red blood cells in microscopic images of blood smears. Dyna, 83(198), 187-194.
CrossRef - Mohammed, E. A., Mohamed, M. M., Far, B. H., & Naugler, C. (2014). Peripheral blood smear image analysis: A comprehensive review. Journal of Pathology Informatics, 5(1), 9.
CrossRef - Mayrose, H., Niranjana, S., Bairy, G. M., Edwankar, H., Belurkar, S., & Saravu, K. (2021, July). Computer Vision Approach for the detection of Thrombocytopenia from Microscopic Blood Smear Images. In 2021 IEEE International Conference on Electronics, Computing and Communication Technologies (CONECCT) (pp. 1-5). IEEE.
CrossRef - Kovalenko, S., Kovalenko, S., Mikhnova, O., Kovalenko, A., Pelikh, D., & Severin, V. (2023, October). An Approach to Blood Cell Classification Based on Object Segmentation and Machine Learning. In 2023 IEEE 4th KhPI Week on Advanced Technology (KhPIWeek) (pp. 1-6). IEEE.
CrossRef - Firoz, R., Ali, M. S., Khan, M. N. U., Hossain, M. K., Islam, M. K., & Shahinuzzaman, M. (2016). Medical image enhancement using morphological transformation. Journal of Data Analysis and Information Processing, 4(1), 1-12.
CrossRef - Mousavi, S. M. H., & Lyashenko, V. (2017, November). Extracting old persian cuneiform font out of noisy images (handwritten or inscription). In 2017 10th Iranian Conference on Machine Vision and Image Processing (MVIP) (pp. 241-246). IEEE.
CrossRef - Mittal, A., Dhalla, S., Gupta, S., & Gupta, A. (2022). Automated analysis of blood smear images for leukemia detection: a comprehensive review. ACM Computing Surveys (CSUR), 54(11s), 1-37.
CrossRef - Mousavi, S. M. H., Victorovich, L. V., Ilanloo, A., & Mirinezhad, S. Y. (2022, November). Fatty Liver Level Recognition Using Particle Swarm optimization (PSO) Image Segmentation and Analysis. In 2022 12th International Conference on Computer and Knowledge Engineering (ICCKE) (pp. 237-245). IEEE.
CrossRef - Uchqun o‘g‘li, B. S., Valentin, L., & Vyacheslav, L. (2023). Pre-processing of digital images to improve the efficiency of liver fat analysis. Multidisciplinary Journal of Science and Technology, 3(1), 107-114.
- Fitri, Z. E., Purnama, I. K. E., Pramunanto, E., & Pumomo, M. H. (2017, August). A comparison of platelets classification from digitalization microscopic peripheral blood smear. In 2017 International Seminar on Intelligent Technology and Its Applications (ISITIA) (pp. 356-361). IEEE.
CrossRef - Dey, R., Roy, K., Bhattacharjee, D., Nasipuri, M., & Ghosh, P. (2015, September). An automated system for segmenting platelets from microscopic images of blood cells. In 2015 International Symposium on Advanced Computing and Communication (ISACC) (pp. 230-237). IEEE.
CrossRef - Lazuardi, L., Sanjaya, G. Y., Candradewi, I., & Holmner, Å. (2013). Automatic platelets counter for supporting dengue case detection in primary health care in indonesia. In 14th World Congress on Medical and Health Informatics (MEDINFO), AUG 20-23, 2013, Copenhagen, DENMARK (pp. 585-588). IOS Press.
- Liu, R., Ren, C., Fu, M., Chu, Z., & Guo, J. (2022). Platelet Detection Based on Improved YOLO_v3. Cyborg and Bionic Systems (Washington, DC), 2022, 9780569-9780569.
CrossRef - Lee, S. J., Chen, P. Y., & Lin, J. W. (2022). Complete blood cell detection and counting based on deep neural networks. Applied Sciences, 12(16), 8140.
CrossRef - Atmanto, Y. K. A. A., Abdullah, A. A., Muhadi, D., & Arif, M. (2022). Determination of Platelet Count Estimation Factor on Peripheral Blood Smear Confirmation Using Field Number 22 Microscope. Indonesian journal of clinical pathology and medical laboratory, 29(1), 24-28.
CrossRef - Drałus, G., Mazur, D., & Czmil, A. (2021). Automatic detection and counting of blood cells in smear images using retinanet. Entropy, 23(11), 1522.
CrossRef - Briggs, C., Harrison, P., & Machin, S. J. (2007). Continuing developments with the automated platelet count 1. International journal of laboratory hematology, 29(2), 77-91.
CrossRef - Harrison, P., Ault, K. A., Chapman, S., Charie, L., Davis, B., Fujimoto, K., … & International Society of Laboratory Hematology Task Force for the Reference Platelet Count. (2001). An interlaboratory study of a candidate reference method for platelet counting. American journal of clinical pathology, 115(3), 448-459.
CrossRef - Mohamed-Rachid, B., Raya, A. F., Sulaiman, A. H., & Salam, A. K. (2015). Comparative analysis of four methods for enumeration of platelet counts in thrombocytopenic patients. Journal of Applied Hematology, 6(3), 119-124.
CrossRef - Wang, Z., Jin, X., Wang, S., You, Q., Wang, J., & Xu, D. (2023). Selection of Automated Platelet Counting Methods Based on Mean Platelet Volume (MPV). Clinical Laboratory, 69(5), 906.
CrossRef