Manuscript accepted on :12-10-2023
Published online on: 23-01-2024
Plagiarism Check: Yes
Reviewed by: Dr. Noora Thamer Al-Dabbagh and Dr. Audrey Jacob
Second Review by: Dr. Arif Ansori
Final Approval by: Dr. Ian James Martin


Rinku Garg1*, Amanpreet Kaur Sandhu1
, Bobbinpreet Kaur2, Bhawna Goyal2
and Ayush Dogra3
1University Institute of Computing, Chandigrah University,Mohali,140413, India.
2Department of Electronics and Communication Engineering, Chandigrah University,Mohali, India.
3Chitkara University Institute of Engineering and Technology, Chitkara University, Punjab, India.
Corresponding Author E-mail: ayush123456789@gmail.com
DOI : https://dx.doi.org/10.13005/bpj/2861
Abstract
The traditional method of studying and diagnosing diseases in plants relies primarily on human vision, which is ineffective in identifying diseases in the plants. The color of the leaves gets changed and develops spots such as yellow, brown and black patches as a result of the symptoms. Manually observing leaves for detection is employed to identify the disease, which takes more time, is more costly, and is less accurate. As a result, use of image processing techniques may be a better alternative than certain other old traditional approaches for speedy and exact illness identification. The symptoms may be seen on plant components such as the fruit, leaves, stems, or lesions. The target is to appropriately identify and categorize the diseases based on the leaf photographs. The processes involved in the procedure include image pre-processing, segmentation, feature extraction, and identification. Bacterial, viral, fungal, and insect-borne diseases are all considered. Mango leaves include anthracnose, bacterial canker, and black sooty mold. In this article, a hybrid filter was proposed based on image enhancement i.e., denoising, reducing blurriness and edge sharping of the images and then segmentation done by taking leaves of these three diseases and results are saved.
Keywords
Denoising; Image Enhancement; Image segmentation; Mango diseases; Precision; Research
Download this article as:
Copy the following to cite this article: Garg R, Sandhu A. K, Kaur B, Goyal B, Dogra A. Design of Filtration Approach for Image Quality Improvement in Mango Leaf Disease Detection and Pharmaceutical Treatment. Biomed Pharmacol J 2024;17(1). |
Copy the following to cite this URL: Garg R, Sandhu A. K, Kaur B, Goyal B, Dogra A. Design of Filtration Approach for Image Quality Improvement in Mango Leaf Disease Detection and Pharmaceutical Treatment. Biomed Pharmacol J 2024;17(1). Available from: https://bit.ly/42gKwwj |
Introduction
The main issues that directly reduces the quality of agricultural production is plant diseases. They influence plants by obstructing a several activities, that includes development of fruits and flowers and photosynthesis, development and growth of plants, and cell division and enlargement.
Various ailments such as fungi, bacteria, phytoplasma, viruses, nematodes, and other organisms can cause plant diseases.1 The degree of diseases brought on by these infections can range from minor symptoms to major like plant death, that depends on the pathogen’s aggressiveness, the host’s resistance, the climatic circumstances, the length of the infection, and other factors. Depending on the pathogen and the affected component, symptoms of plant diseases can include spots on the leaves, leaf blights, root rots, fruit rots, spots on the fruit, wilt, dieback, and decline.2 Both the production costs and the revenues of agricultural stakeholders are adversely affected by these losses. Unfortunately, methods for rapid and precise identification are still limited. A nation’s food supply and nutritional security, as well as farmer welfare and livelihoods, are all at risk if disease epidemics take place.
The identification and categorization of plant lesions are the primary responsibilities for improving the quality of plant output for economic growth. Farmers use their eyes to identify whether a leaf is diseased. This technique is unstable, inconsistent, and prone to errors. Many studies on deep learning algorithms for identifying leaf diseases have been proposed.3 Several strategies for detecting and classifying diseases in mango leaves were described in this article. Safeguarding plants against severe diseases by learning to detect signs and practicing preventive. A comprehensive approach begins with identifying the pathogen. Then, choose a therapy approach that is safe, effective, and responsible.4-6
Mango has been cultivated for 4,000 years and is a native of Southeast Asia and India. There are numerous varieties of mangoes, each with a unique flavor, appearance, size, and color. This fruit is not only tasty, but it also has a remarkable nutritional profile. Mango and its nutrients provide several health advantages, including increased immunity and intestinal health. Mango crop plagued by different diseases, resulting in farmer losses and a negative impact on the country’s economy. Mango producers in Karnataka’s Kolar district7, dropped numerous quintals of mango in 2021 owing to the outbreak of Anthracnose mango disease, causing in a price decline and loss of farmers’ revenue. In 2022, a fungal disease affected mango blooms, resulting in a 10% production reduction in Coimbatore.8 Nonetheless, the diseases detection method stays consistent across all techniques. To do this, the images should go through the following four main procedures: pre-processing, segmentation, feature extraction, and classification. The accuracy of mango leaf disease detection using image processing depends on the quality of the acquired images, the effectiveness of the preprocessing techniques used, and the selection of appropriate features and algorithms. Therefore, it is important to carefully design and evaluate each step of the process to ensure accurate and reliable disease detection. The preprocessing stage can be used in agricultural sciences to build a system based on statistical analysis and blob detection that can identify and categorize the many types of diseases. The segmentation strategy includes splitting an image into several sections with distinct meanings for locating the mango leaves diseases areas and this is the primary technique for extracting image information.9 The important image characteristics represent texture, shape, and color-related aspects and then classifiers are used to predicting the type of mango disease.10 The objective of this work is to enhance the image quality which in turn can improve the classification accuracy various domain filters are deployed to achieve high performance validated through quality metrics. The arrangement of the remaining portion of this article is as follows: Sect. 2 discusses the various techniques of image processing used by other authors, Sect. 3 discusses different diseases and their symptoms exits in mango leaves, Sect. 4 includes methods for mango leaf diseases detection, Sect. 5 discusses preprocessing filters, Sect. 6 have proposed methodology, Sect. 7 discusses the metrics used to measure the performance, Sect. 8 includes experimental setup, Sect. 9 covers all results of the investigation, Sect. 10 will discusses the summary of the article.
Literature Review
Several researchers are working towards design and development of automated systems for mango diseases detection. The necessity and importance of the pre-processing stage to improve the accuracy of complete system is still a gap for future work. The related issues and existing work are discussed below.
Preprocessing
Pre-processing is done to improve the image’s quality so we can study it more effectively. With pre-processing, helps in reducing unwanted distortions and improve some qualities that are essential for the application that needs to create. Depending on the use, the qualities of the images may vary. The Fourier transform, geometric transformations, picture filtering and segmentation, image enhancement, and pixel brightness transformations and brightness corrections are a few examples of image pre-processing techniques.
The model performance11 verified using real-world images and a non-destructive manner. The image was pre-processed using histogram equalization, which may equalize the consistency in the collected images. The center square crop technique was used to downsize each image for investigation. For mango disease identification, the built-in CNN-based classification model was trained and estimated.12 It obtained an input image in the first phase, and then preprocess it by turning it into a grayscale image in the second phase. To create the final output image, segmentation is lastly done to the preprocessed image while considering intensity, area, and perimeter. Then to determine the percentage of the area afflicted by anthracnose, the information is added up together.
Segmentation
Image segmentation is next step for processing digital images and to analyze for separating various portions or parts of the image based on the image pixels characteristics. A crucial stage in many image processing and computer vision applications, such as object identification, tracking, recognition, and analysis, is image segmentation. The task at hand and the characteristics of the image being segmented influence the method of image segmentation that is used.
The leaf images13 were upgraded using a hybrid technique that combines a 3D-Gaussian filter, a 3D-median filter, de-correlation, and a 3D-box filter. A strong correlation-based method is used to segment the lesion areas into discrete groups, and the results are improved by incorporating expectation maximization (EM) segmentation. Finally, using comparison-based parallel fusion, the color, color histogram, and local binary pattern (LBP) features are combined. The retrieved characteristics are optimized using a genetic procedure and categorized using a One M-SVM as compared to All M-SVM.
The Object Evolution Mapping segmentation model, created14, that has been used plant leaf disease images. The image segmentation technique connects related pixels and object-growing pixels. The matrix is formed containing each pixel weight and iterated using the Euclidean distance metric. The results show that the suggested model is superior at segmenting disease regions that display color, texture, and shape change.
Feature extraction
Leaf image feature extraction involves extracting meaningful information from leaf images that can be used for various purposes such as plant identification, disease detection, and crop yield prediction. Feature extraction is the process of converting unprocessed raw data into numerical feature-based data that can be processed while preserving the information from the original data set. Compared to directly applying machine learning to raw data, it yields better results.
The four varieties of leaves15 were collected: Anthracnose, Gall midge, Powdery mildew, and healthy leaves. High resolution images are used to detect tiny disease blobs, and contrast enhancement is applied in pre-processing. A wrapper-based feature selection technique was used to pick measurement-based features from the blobs and used ANN to identify sickness early on, and the findings are compared to other CNN models like as (AlexNet, VGG16, ResNet-50). ANN outperforms CNN (89.41% versus 78.64%, 79.92%, and 84.88%, respectively), demonstrating that these approaches can be applied on low-end devices like as smart phones.
There was no need for segmentation16 was showed because CNN uses deep neural networks, which can extract features. The stochastic gradient descent (SGD) methodology is replaced with the optimizer Adam to determine the weights in this model. Root Mean Square Propagation (RMSProp), an extension of the gradient-based optimization technique known as Adaptive Gradient Descent (AdaGrad), is used to normalize the gradients. By integrating the finest attributes of these two optimizers, the Adam optimizer provides a superior iteration of the optimization approach that can manage sparse gradient on noisy issues.
Classification
Categorizing the image is removing information classes from a multiband raster image. The raster data produced by image classification can be used to create themed maps. After feature extraction, machine learning or computer vision algorithms can be used to classify the different diseases present in the mango leaf image. Decision trees, neural networks, and support vector machines (SVM) are a few typical methods used in disease categorization.
MCNN17 was used to identify Anthracnose, a fungal illness. It made use of real-time images collected at Shri Mata Vaishno Devi University (1070 images). It used CNN models to compare the work of other writers on different plants. Its pre-processing includes contrast improvement, scaling, and splitting photos across the training and testing datasets. In comparison to other technologies, it had a performance better than others.
SVM and ANN18 were used classifiers to test the extracted and selected features from the images that are segmented using proposed technique that automatically detect and classify the leaf diseases.
Vein pattern22 has used for the leaves to separate the diseased parts of the leaf. The characteristics were then extracted using a fusion method based on canonical correlation analysis (CCA), and the results were tested and verified using a cubic SVM, which had higher accuracy than previous models.
Table 1: Techniques used for image processing process
Phases |
Technique |
Reference |
Remarks |
Pre-processing |
Contrast Enhancement
|
Early Disease Classification of Mango Leaves Using Feed-Forward Neural Network and Hybrid Metaheuristic Feature Selection15, Machine Learning Algorithm Development for detection of Mango infected by Anthracnose Disease11, Mango Diseases Identification by a Deep Residual Network with Contrast Enhancement and Transfer Learning24 |
Contrast enhancement of leaf images can be a useful technique to improve the visibility and quality of images. But it may lead to loss of information, noise amplification, artifact generation and colour distortion. |
Histogram equalization |
Multilayer Convolution Neural Network for the Classification of Mango Leaves Infected by Anthracnose Disease17, Classification of Mango Leaves Infected by Fungal Disease Anthracnose Using Deep Learning16, Machine Learning Algorithm Development for detection of Mango infected by Anthracnose Disease11 |
For improving image contrast, a common technique is histogram equalisation. It involves parameters that influence the degree of enhancement. Selecting appropriate parameters can be subjective and dependent on the specific image. Improper parameter settings may lead to unsatisfactory results. |
|
Noise removal |
Multilayer Convolution Neural Network for the Classification of Mango Leaves Infected by Anthracnose Disease17, Mango leaf disease recognition using neural network and support vector machine9 |
It reduces unwanted noise in leaf images, but they also lead to loss of fine details, blurring or smudging of edges and over smoothing and loss of natural appearance. |
|
Edge enhancement |
Mango leaf disease identification using fully resolution convolutional network25, Mango Disease Detection by Using Image Processing26 |
It enhances the sharpness and visibility of edges in leaf images. It leads to amplification of noise, artifact generation and overemphasis of existing features. |
|
Segmentation |
Thresholding technique |
Hybrid approach for anthracnose detection using intensity and size features 12, Mango Disease Detection by Using Image Processing26 |
It is used to separate foreground objects, such as leaves, from the background in an image based on intensity values. It requires the selection of an appropriate threshold value to differentiate the foreground and background. Choosing an optimal threshold value is challenging. |
Edge based |
Multilayer Convolution Neural Network for the Classification of Mango Leaves Infected by Anthracnose Disease17, Mango leaf disease identification using fully resolution convolutional network25 |
To identify boundaries or edges of leaves, in an image. It involves the selection of parameters, such as edge detection thresholds or smoothing parameters, which can significantly impact the segmentation results. Choosing appropriate parameter values can be challenging. |
|
Region based |
Mango leaf disease recognition using neural network and support vector machine9, Mango Disease Detection by Using Image Processing26 |
To partition an image into coherent regions based on certain criteria. It relies on user-defined parameters, such as thresholds or similarity measures, to define and separate regions. Selecting appropriate parameter values can be challenging. |
|
Feature Extraction |
Statistics |
Early Disease Classification of Mango Leaves Using Feed-Forward Neural Network and Hybrid Metaheuristic Feature Selection15, Mango leaf disease identification using fully resolution convolutional network25, Deep Learning for Image Based Mango Leaf Disease Detection27 |
It derives meaningful features from leaf images by analysing statistical properties, such as mean, variance, or histogram. |
Geometry |
Early Disease Classification of Mango Leaves Using Feed-Forward Neural Network and Hybrid Metaheuristic Feature Selection15, Mango leaf disease recognition using neural network and support vector machine9, Mango leaf disease identification using fully resolution convolutional network25, Mango Disease Detection by Using Image Processing26 |
It extracts features from leaf images based on geometric properties, such as shape, size, or spatial relationships. |
|
Texture |
Early Disease Classification of Mango Leaves Using Feed-Forward Neural Network and Hybrid Metaheuristic Feature Selection15, Hybrid approach for anthracnose detection using intensity and size features12, Mango Disease Detection by Using Image Processing26 |
It captures the textural patterns and characteristics of leaf images. |
Type of Mango Diseases
Following are the most found diseases and their symptoms in the all type of mango trees.
![]() |
Table 2: Mango leaf diseases and their symptoms. |
Mango leaf disease detection methods
Various steps required to process and detecting diseases from mango leaf images are shown in fig1.
Techniques for Pre-Processing
Following are the various techniques for enhancing the features of image:
Contrast enhancement techniques is to make things in the image more visible, it modifies the objects’ relative brightness and blackness. Using a Gray-level transform to translate the image’s grey levels30 to new values allows one to change an image’s contrast and tonality.
An image’s noise can be reduced or eliminated using a noise reduction algorithm. The entire image is smoothed out by the noise reduction algorithms, leaving only the edges of contrast. This lessens or eliminates noise visibility. However, these techniques might obscure minute, low-contrast details.31 Various sounds have distinct qualities that make them distinguished from one another.
The issue of image denoising can be expressed mathematically as follows:

in equation 1, y is the observed noisy image, x is the unknown clean image, and ƞAWGN stands for additive white Gaussian noise (AWGN) with a standard deviation of n. The median absolute deviation, block-based estimation, and PCA-based methods can all be used in practical applications to estimate this noise. Noise reduction aims to lower noise in natural pictures (SNR) to increase the signal-to-noise ratio and lessen the loss of original information. The major challenges for denoising the image are as follows:
Smooth the flat surfaces
Blurriness should not be there while preserving the edges
Textures ought to be kept
No new artefacts should be produced
Edge enhancement is a form of image processing filter that increases contrast at an image’s edges to heighten its apparent sharpness (acutance). The filter boosts visual contrast in the area right next to sharp edge borders of an image35, such as the border between a subject and a background with contrasting colours.
Histogram is a computer image processing called equalisation enhances picture contrast. This is achieved by spreading out the most common intensity values uniformly and thereby expanding the image’s intensity range.
![]() |
Figure 1: Steps for leaf image processing. |
Techniques for segmentation
To make an image easier to analyse, we can change its pixels using the image segmentation technique38 known as thresholding. The thresholding technique transforms a colour or grayscale image into a binary image, which only comes in black and white.
Edge-based segmentation is used to identify the edges in a picture, it employs a variety of edge detection operators. These edges signify visual breaks in colour, texture, and other elements like texture and colour. As we move from one area to another, the degree of grey may change. Hence, we can find the edge if we can find the discontinuity.
There is region-based segmentation; for a pixel to be classified into similar pixel areas, certain predetermined rules must be met by the pixel. In the case of a noisy image, region-based segmentation approaches are recommended above edge-based segmentation techniques.
Various feature extraction
In statistically based feature selection approaches39, the relationship between each input variable and the target variable is statistically analysed, and the input variables with the strongest association to the target variable are chosen.
Anything that demonstrates geometric features, such as points, lines, curves, or surfaces, is referred to as having geometric aspects. Utilising feature detection techniques, several characteristics can be discovered, including corner features, edge features, blobs, ridges, noticeable spots, picture texture, and others.
Texture is a property that is used to divide and categorise pictures into regions of interest. By arranging colours or intensities spatially, texture adds information to a picture. The texture of a neighbourhood is determined by the spatial distribution of intensity levels.
Colour-based features: These features are derived from the colour distribution of the leaf, such as mean colour, colour histogram, and colour moments. Colour-based features are useful for identifying leaves with distinct colour patterns or for detecting colour variations due to diseases or environmental factors.
Challenges
The pre-processing phase increases the visibility of the disease area in relation to the actual image. Various problems of the pre-processing techniques are (a) to remove the noise from leaf covered with noisy background (b) extracting the optimal contrast between the background and covering of the leaf; (c) modifications in lighting and variation40 (d) values of a source image with low intensity; and (e) with its hundreds of different wavelength bands, a tremendous number of associated redundancies.
The primary goal of picture segmentation in agriculture is to segregate images should have the sickness and background. There are several difficulties with segmenting the diseased section of the image, such as (a) When using colour-based segmentation, the colour of the disease can change.; (b) Colour fluctuation makes the segmentation operations challenging; (c) alterations in the illumination; (d) size of disease area gets changed; (e) quantity of fruit; and (f) region-growing segmentation method, which takes a long time to process. These issues reduce the system’s overall effectiveness and influence how accurately diseases are detected.
Various pre-processing filters
Gaussian blur
The commonly used image denoising method known as the GF (gaussian filter) is based on a Gaussian function. The Gaussian function is a bell-shaped curve that describes the distribution of values in an image. The Gaussian function (GF) performs a convolution operation on the image, replacing each pixel with a weighted average of its neighbours, the weights of which are specified.
The formula for the Gaussian function is:

Where σ is the standard deviation of the Gaussian distribution and x and y are the distances from the centre pixel.
The GF has several advantages over other image denoising techniques. It preserves edges and fine details in the image while removing noise, and it is computationally efficient and easy to implement. However, certain types of noise, including salt-and-pepper noise, cannot be removed with this method, and if the standard deviation is too large, the image may become blurry.
Median filter
A most liked nonlinear digital filtering method for eliminating noise is the median filter (MF). Because it may maintain edges while reducing noise, MF is frequently employed in digital image processing. The main idea behind the MF is to repeatedly replace each element in the signal with the median of the entries that are closest to it.41 The MF can remove the impact of input noise values with exceptionally large magnitudes. The median of the input values corresponding to the moments just before t is used to determine the output z of the MF at moment t, as shown in equation 3:

Non local means filter
It is an image pre-processing algorithm for image denoising. While filtering an image, all pixels are averaged and weighted according to how similar they are to the target pixel. And produces significantly less loss of detail in the image during post-filtering.42
The NLMF (non-local means filter) is described as follows:
Weighting function is computed from mean with normal distribution u=B(p) and standard deviation.

Where h is standard deviation (filtering parameter) and B(p) is mean value around point p.
Then factor i.e., C(p) for normalizing obtained from

Now, u(p) in image is calculate filtered value at point p where p and q are the points in the image having Ω as the image area

And, v(p) at any point q is unfiltered value, f(p,q) is the weighted function for which integral is calculated
Bilateral filter
It is a denoising filter that smooths an input image while keeping its edges intact. A weighted average of its neighbours replaces each pixel.43 Each neighbour’s weight is determined by a spatial component that penalises distant pixels and a range component that penalises pixels with varying intensities.
The weights of this filter are based on the spatial and intensity distances, and it computes a weighted sum of the pixels in a limited area as shown in fig 2. Edges are nicely kept while noise is averaged out in this manner. The output of the BF (bilateral filter) is determined mathematically at pixel position x as follows:

where σd and σr are factors affecting how weights in the spatial and intensity domains fall off, respectively, and C is the normalising constant, and the spatial region surrounding x in as in equation 8:

![]() |
Figure 2: The bilateral filter keeps the edges of an input image while smoothing it out. |
The restoration of images that had been distorted by the combination of impulse and Gaussian noise performed better using this noise reduction algorithm.47-49
High Boost filter
Image should be enhanced before moving to further steps. The major goals of the enhancement process are low frequency components and higher frequency pixels. It is a technique for sharpening that emphasises high-frequency components that represent the finer details of the image without erasing low-frequency components. It will enhance the image’s high frequency elements. High frequency components are sharpened by subtracting the original image from a low pass filtered, smoothed image.
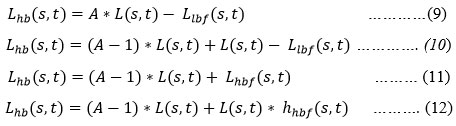
Where L(s,t) is the original picture, Llpf(s,t) is the low pass filtered image, and Lhpf(s,t) is the high pass filtered image, A is a factor influencing weights also known as the amplification factor.
Edge sharpening and noise reduction must be accomplished simultaneously; therefore, the proper amplification factor must be selected when creating a HBF (high boost filter). Producing as many sharp edges and as little noise as feasible is the objective function’s aim.
Proposed Methodology
In this paper, a hybrid filter strategy for enhancing the image quality based on bilateral and spatial filters is proposed. Noise removal is achieved by deploying suitable denoising filter and edge enhancement is achieved by deploying HBF.
![]() |
Figure 3: Proposed Methodology |
Fig. 3 gives the thorough explanation of the proposed algorithm’s many stages. The input image is a mango diseased leaf image then patch from image is selected and coloured image is converted to double precision then both are combined to produce denoised image using denoising filter with degree of smoothing value 4. The HBF is used to sharpen these denoised images. The use of HBF reduces noise, allowing the subsequent phases of our suggested process to operate more effectively. We tested our method using different Structuring element sizes and forms, and the resulting image was subjectively and objectively assessed for quality assurance.
Input: IRGB.
Noise Detection
Resize IRGB to equal dimensions.
Convert the image IRGB to double precision (IDRGB).
Extract the patch IP from image with clear intensity.
Noise Reduction
For each noisy pixel in IDRGB
Compute the variance σ² in from the origin X.
Set the Degree of Smoothing value =4 more than the patch variance.
Display the denoised image IX.
Compute IPSNR of IX.
Edge Sharpening
Select the size of filter h_size 2-element vector of positive integers.
Select the value of sigma i.e., 0.5.
Select value of Amplification factor A=2
Calculate GF for h_size and sigma value.
Apply GF to IX to get IGLBF.
Now subtract IGLBF from IX to get IHPF.
Calculate IHFBF= (A-1) * IX + IX – IGLBF
Calculate PSNR of IHFBF image.
Metrics of Performance
The proposed approach for picture denoising was objectively validated using various image-enhancement quality indicators.44 The significance of these actions supported the accuracy of both the information included in the image and its overall quality.
PSNR (peak signal-to-noise ratio).
More Value indicates the better strength of image over noise and hence better enhancement.

MSE (Mean Squared Error)
Depicts error value between images, Lower the error better is the enhancement. A trade-off value needs to maintain in case image sharpening is applied.

Experimental Setup
MATLAB R2021a is used to implement the specified methodology, with an Intel Core i3 running at 2.10 GHz and 4.00 GB of RAM. For the implementation and evaluation of suggested algorithms, numerous images from the mango leaf disease image collection45 showing various plant diseases, including anthracnose, black sooty mould, and bacterial canker, are used. All the photographs obtained have 256 * 256 dimensions and a resolution of 72 dpi. The data set28-29 contains 525,656 and 500 photos, respectively, of the disease’s anthracnose, black sooty mould, and bacterial canker. The degree of smoothing mask is determined with weight w=4 for denoising filtering. And then denoised images are sharpened with edge enhancement filter.
Results and Discussion
The proposed methodology’s performance was evaluated using a mango disease dataset.46 The system’s effectiveness was evaluated using four-four images of each disease like bacterial canker, anthracnose, and black sooty mould. The experiment was carried out with various degrees of smoothing for extracted patches with no sharp edges. Following the experiment, it was discovered that the greatest results were obtained with a degree of smoothing ‘4’ as opposed to other filters. This will aid in producing the same result regardless of the orientation angle of an object in a scenic photograph with respect to the sample grid. As we scan over the tabular numbers, our proposed approach with a degree of smoothing of ‘4’ yields the best values for the objective measure as shown in table III and IV. The performance degrades as the value of degree of smoothing increases. And for the same images MSE also calculated which shows very less amount of error mostly 0.00 in almost all images.
Afterwards the images were selected with proposed degree of smoothing for segmentation which will do coloured segmentation for separating different parts of the leaves and results are shown in table V. Then RGB feature extraction that are type of colour-based feature extraction technique that involves analysing the red, green, and blue colour channels of a leaf image separately. Features are Colour moments that are statistical measures that describe the distribution of pixel intensities for each colour channel. Common colour moments include mean, median, standard deviation, and range as shown in table VI. By computing colour moments for each colour channel, extracted features that describe the colour distribution and texture of the leaf. Same images are denoised using others filters like median filter and non-local means filter, gaussian filter and PSNR calculated for both and obtained results are compared with proposed filter with degree of smoothing as 4 which gives better results that can be seen in table 3.
Table 3: Results of PSNR and MSE after applying median filter, non-local means filter, gaussian filter, bilateral filter, and proposed filter
Input |
|
PSNR |
MSE |
|||
Median filter |
Non local means filter |
Gaussian Blur with smoothing=0.5 |
Bilateral filter with deg. Of smoothing=16 |
Proposed filter with Deg. Of smoothing=4 |
||
Anthracnose |
51.8964 |
53.7218 |
60.0363 |
59.3945 |
63.4375 |
0.0000 |
46.3806 |
61.1851 |
53.1527 |
56.6236 |
61.7590 |
0.0000 |
|
48.8606 |
53.6191 |
55.7573 |
54.4195 |
57.6851 |
0.0000 |
|
47.1974 |
62.0802 |
53.7625 |
69.0887 |
78.1281 |
0.0000 |
|
Black sooty mould |
40.3253 |
33.4188 |
44.7639 |
42.6473 |
44.9048 |
0.0001 |
35.6404 |
31.3380 |
43.1613 |
52.5436 |
59.1552 |
0.0001 |
|
36.3824 |
28.9621 |
40.6723 |
38.6957 |
41.0597 |
0.0002 |
|
45.3705 |
45.4102 |
52.1514 |
54.6762 |
58.4421 |
0.0001 |
|
Bacterial canker |
37.8429 |
38.4572 |
45.3290 |
43.5951 |
46.5263 |
0.0001 |
36.3520 |
34.9378 |
37.1222 |
36.8739 |
39.9890 |
0.0002 |
|
37.9163 |
37.3863 |
43.2477 |
41.7760 |
43.9275 |
0.0001 |
|
37.6454 |
39.1372 |
44.3805 |
45.2263 |
48.7944 |
0.0001 |
The images must be sharpened and denoised in order to retrieve the region of interest accurately and crisply for use in later phases like segmentation, classification, and so on. For illness identification, the diseased area needs to have distinct boundaries. With the use of high-boost filtering, noise was removed and object boundaries were clearly defined.
![]() |
Table 4: Denoised images of Anthracnose, Sooty Mold, and Bacterial canker with different filters |
Above denoised images are segmented and results are shown in table 5.
![]() |
Table 5: Segmentation results of denoised images. |
Then following RGB features extracted for above segmented images as shown in table 6.
Table 6: Extracted RGB features of segmented images
Diseases |
Colour |
Mean |
Median |
Standard Deviation |
Range |
Anthracnose -1 |
R |
34.65 |
0.00 |
77.99 |
255 |
G |
54.31 |
0.00 |
98.26 |
255 |
|
B |
50.19 |
0.00 |
91.88 |
255 |
|
Anthracnose -2 |
R |
57.13 |
4.00 |
90.28 |
255 |
G |
76.06 |
19.00 |
93.20 |
255 |
|
B |
99.53 |
36.00 |
104.27 |
255 |
|
Anthracnose -3 |
R |
40.66 |
1.00 |
78.62 |
255 |
G |
42.63 |
1.00 |
76.16 |
255 |
|
B |
31.41 |
0.00 |
67.63 |
255 |
|
Bacterial canker -1 |
R |
16.36 |
0.00 |
51.81 |
255 |
G |
41.48 |
0.00 |
84.51 |
255 |
|
B |
41.54 |
0.00 |
84.10 |
255 |
|
Bacterial canker -2 |
R |
14.95 |
0.00 |
49.18 |
255 |
G |
9.60 |
0.00 |
38.55 |
255 |
|
B |
29.75 |
0.00 |
71.69 |
255 |
|
Bacterial canker -3 |
R |
18.22 |
0.00 |
56.42 |
255 |
G |
27.32 |
0.00 |
69.41 |
255 |
|
B |
27.39 |
0.00 |
69.25 |
255 |
|
Black sooty mould -1 |
R |
47.05 |
2.00 |
86.46 |
255 |
G |
42.92 |
0.00 |
87.25 |
255 |
|
B |
42.89 |
0.00 |
87.15 |
255 |
|
Black sooty mould -2 |
R |
72.07 |
9.00 |
98.80 |
255 |
G |
75.85 |
5.00 |
100.50 |
255 |
|
B |
63.96 |
6.00 |
93.19 |
255 |
|
Black sooty mould -3 |
R |
36.73 |
3.00 |
65.45 |
255 |
G |
96.87 |
19.00 |
110.48 |
255 |
|
B |
86.14 |
17.00 |
99.02 |
255 |
Conclusion
In this paper, a hybrid filter for image enhancement in terms of denoising filter with best smoothing level 4, edge enhancement with HBF have been proposed and segmentation based on spatial domain filtering terms of image enhancement.
Using images of mango diseased images, the algorithm was tested, and significant gains in PSNR and other quality metrics were made. Proposed hybrid filter computes PSNR between 40dB to 80dB as compared to MF. NLMF, GF and BF (35dB to 50dB), (25dB to 60dB), (35dB to 60dB) and (35dB to 70dB) respectively. Further the proposed method yields MSE range from 0.0000 to 0.0002. The HBF sharpens the edges while the denoising filter lowers noise in the input images. Twelve photos were used to test out structure elements of various sizes and forms. The results demonstrate that the suggested strategy considerably enhances image quality and has the potential to be useful for later stages of disease detection. These enhanced images are used further for segmentation and RGB features were extracted for the same enhance segmented images. Our future work will be toward deployment of this method for achieving robust segmentation of lesions present in mango leaves. This can improve overall classifier accuracy for mango leaf disease detection. In future this work can be extended to test the accuracy of the complete detection system for different environmental system.
Acknowledgement
None
Conflicts of Interest
The authors affirm that they do not have any competing interests.
Funding sources
No funding provided by any authority.
References
- C. Gupta, V. K. Tewari, R. Machavaram, and P. Shrivastava, “An image processing approach for measurement of chili plant height and width under field conditions,” J. Saudi Soc. Agric. Sci., vol. 21, no. 3, pp. 171–179, 2022.
CrossRef - S. Thenmozhi, R. J. Lakshmi, M. V Kumudavalli, I. Ibrahim, and R. Mohan, “A Novel Plant Leaf Ailment Recognition Method using Image Processing Algorithms,” J. Sci. Ind. Res. (India)., vol. 80, no. 11, pp. 979–984, 2021.
CrossRef - J. G. A. Barbedo, L. V Koenigkan, and T. T. Santos, “Identifying multiple plant diseases using digital image processing,” Biosyst. Eng., vol. 147, pp. 104–116, 2016.
CrossRef - N. Ganatra and A. Patel, “A multiclass plant leaf disease detection using image processing and machine learning techniques,” Int. J. Emerg. Technol., vol. 11, no. 2, pp. 1082–1086, 2020.
- K. Anitha, G. Keerthiga, and A. Hema Mailini, “Plant health monitoring system through image processing and defects overcoming through embedded system,” Int. J. Recent Technol. Eng., vol. 8, no. 1, pp. 406–408, 2019.
- I. Philipp and T. Rath, “Improving plant discrimination in image processing by use of different colour space transformations,” Comput. Electron. Agric., vol. 35, no. 1, pp. 1–15, 2002.
CrossRef - https://economictimes.indiatimes.com/news/economy/agriculture/price-crash-and-fungal-infection-force-growers-to-dump-quintals-of-mangoes-on-highway/articleshow/83891860.cms
- https://www.thehindu.com/news/cities/Coimbatore/fungal-infection-affects-mango-flowers-in-krishnagiri/article65084129.ece
- M. R. Mia, S. Roy, S. K. Das, and M. A. Rahman, “Mango leaf disease recognition using neural network and support vector machine,” Iran J. Comput. Sci., vol. 3, no. 3, pp. 185–193, Sep. 2020.
CrossRef - C. Trongtorkid and P. Pramokchon, “Expert system for diagnosis mango diseases using leaf symptoms analysis,” in 2018 International Conference on Digital Arts, Media and Technology (ICDAMT), 2018, pp. 59–64.
CrossRef - Wongsila, Suwit, Parinya Chantrasri, and Pradorn Sureephong. ” Machine Learning Algorithm Development for detection of Mango infected by Anthracnose Disease.” In 2021 Joint International Conference on Digital Arts, Media and Technology with ECTI Northern Section Conference on Electrical, Electronics, Computer and Telecommunication Engineering, pp. 249-252. IEEE, 2021.
CrossRef - K. Swetha, V. Venkataraman, G. . Sadhana, and R. Priyatharshini, “Hybrid approach for anthracnose detection using intensity and size features,” in 2016 IEEE Technological Innovations in ICT for Agriculture and Rural Development (TIAR), 2016, pp. 28–32.
CrossRef - Khan, Muhammad Attique, M. Ikram Ullah Lali, Muhammad Sharif, Kashif Javed, Khursheed Aurangzeb, Syed Irtaza Haider, Abdulaziz Saud Altamrah, and Talha Akram. “An optimized method for segmentation and classification of apple diseases based on strong correlation and genetic algorithm based feature selection.” IEEE Access 7 (2019): 46261-46277.
CrossRef - Shanmuga Rajathi D, D. Maheswari. “An Image Segmentation Technique -OEM for Plant Leaf Disease.” International Journal of Recent Technology and Engineering (IJRTE) 8 (2020).
CrossRef - T. N. Pham, L. Van Tran, and S. V. T. Dao, “Early Disease Classification of Mango Leaves Using Feed-Forward Neural Network and Hybrid Metaheuristic Feature Selection,” IEEE Access, vol. 8, pp. 189960–189973, 2020.
CrossRef - P. Kumar, S. Ashtekar, S. S. Jayakrishna, K. P. Bharath, P. T. Vanathi, and M. Rajesh Kumar, “Classification of Mango Leaves Infected by Fungal Disease Anthracnose Using Deep Learning,” in 2021 5th International Conference on Computing Methodologies and Communication (ICCMC), 2021, pp. 1723–1729
CrossRef - U. P. Singh, S. S. Chouhan, S. Jain, and S. Jain, “Multilayer Convolution Neural Network for the Classification of Mango Leaves Infected by Anthracnose Disease,” IEEE Access, vol. 7, pp. 43721–43729, 2019.
CrossRef - E. Vamsidhar, P. J. Rani, and K. R. Babu, “Plant disease identification and classification using image processing,” Int. J. Eng. Adv. Technol., vol. 8, no. 3 Special Issue, pp. 442–446, 2019.
- H. M. Yu, J. L. Wang, R. Kang, K. J. Chen, and D. Y. Liu, “Automatic identification of asian rice plant-hopper based on image processing,” Appl. Eng. Agric., vol. 33, no. 5, pp. 591–602, 2017.
CrossRef - J. Vijayakumar and S. Arumugam, “Study of betelvine plants diseases and methods of disease identification using digital image processing,” Eur. J. Sci. Res., vol. 70, no. 2, pp. 240–244, 2012.
- Sandino, J. D., Ramos-Sandoval, O. L., & Amaya-Hurtado, D. (2016). Method for estimating leaf coverage in strawberry plants using digital image processing. Revista Brasileira de Engenharia Agrícola e Ambiental, 20, 716-721.
CrossRef - Saleem, R., Shah, J. H., Sharif, M., Yasmin, M., Yong, H. S., and Cha, J. (2021). Mango Leaf Disease Recognition and Classification Using Novel Segmentation and Vein Pattern Technique. Applied Sciences, 11(24), 11901.
CrossRef - J. Vijayakumar and S. Arumugam, “Odium piperis fungus identification for piper betel plants using digital image processing,” Int. J. Soft Comput., vol. 9, no. 4, pp. 207–212, 2014.
- K. Trang, L. TonThat, N. Gia Minh Thao, and N. Tran Ta Thi, “Mango Diseases Identification by a Deep Residual Network with Contrast Enhancement and Transfer Learning,” in 2019 IEEE Conference on Sustainable Utilization and Development in Engineering and Technologies (CSUDET), 2019, pp. 138–142.
CrossRef - Saleem, R., Shah, J. H., Sharif, M., and Ansari, G. J. (2021). Mango leaf disease identification using fully resolution convolutional network. Comput. Mater. Contin, 69, 3581-3601.
CrossRef - Veling, P. S., Mr Rohit S. Kalelkar, Miss Likhita V. Ajgaonkar, and Miss Nivedita V. Mestry. ”Mango Disease Detection By Using Image Processing.” International journal for research in applied science and engineering technology 7, no. 4 (2019): 3717-3726.
CrossRef - Gulavnai, S., Patil, R. (2019). Deep Learning for Image Based Mango Leaf Disease Detection. International Journal of Recent Technology and Engineering, 8(3S3), 54-56.
CrossRef - https://www.kaggle.com/datasets/mypapit/harumanis-leaves-basic-2021
- https://www.kaggle.com/datasets/annisarizki/mango-leaves-imagerecognition-pa
- Modi, H., Trivedi, N., Soni, P., and Patel, H. (2018). Design and Implementation of Image Processing Techniques for Plant Disease Detection. International Journal of Applied Engineering Research, 13(10), 7724- 7758.
- Binnar, V., Sharma, S. (2023, January). Plant Leaf Diseases Detection Using Deep Learning Algorithms. In Machine Learning, Image Processing, Network Security and Data Sciences: Select Proceedings of 3rd International Conference on MIND 2021 (pp. 217-228). Singapore: Springer Nature Singapore.
CrossRef - M. Olech, L. Komsta, R. Nowak, L. Cieśla, and M. Waksmundzka-Hajnos, “Investigation of antiradical activity of plant material by thin-layer chromatography with image processing,” Food Chem., vol. 132, no. 1, pp. 549–553, 2012.
CrossRef - A. S. Zamani et al., “Performance of Machine Learning and Image Processing in Plant Leaf Disease Detection,” J. Food Qual., vol. 2022, 2022.
CrossRef - Y. Wang, “Evaluation of English translation accuracy of green plant surface irrigation and food words based on image processing,” Arab. J. Geosci., vol. 14, no. 15, 2021.
CrossRef - Manoharan, N., Thomas, V. J., Dhas, D. A. S. (2021, August). Identification of Mango Leaf Disease Using Deep Learning. In 2021 Asian Conference on Innovation in Technology (ASIANCON) (pp. 1- 8). IEEE.
CrossRef - K. S. Patle, R. Saini, A. Kumar, and V. S. Palaparthy, “Field Evaluation of Smart Sensor System for Plant Disease Prediction Using LSTM Network,” IEEE Sens. J., vol. 22, no. 4, pp. 3715–3725, Feb. 2022.
CrossRef - A. D. Mengistu, S. G. Mengistu, and D. Melesew, “An automatic coffee plant diseases identification using hybrid approaches of image processing and decision tree,” Indones. J. Electr. Eng. Comput. Sci., vol. 9, no. 3, pp. 806–811, 2018.
CrossRef - V. Singh and A. K. Misra, “Detection of plant leaf diseases using image segmentation and soft computing techniques,” Inf. Process. Agric., vol. 4, no. 1, pp. 41–49, Mar. 2017.
CrossRef - Tumang, Gina S. ”Pests and diseases identification in mango using MATLAB.” In 2019 5th International Conference on Engineering, Applied Sciences and Technology (ICEAST), pp. 1-4. IEEE, 2019.
CrossRef - Garg, R., Sandhu, A. K. (2022, April). Comparison of Various Proposed Techniques for Processing of Different Images. In 2022 2nd International Conference on Advance Computing and Innovative Technologies in Engineering (ICACITE) (pp. 1028-1032). IEEE.
CrossRef - Qin, F., Jiang, L., Xie, L., Cao, L., Zhu, L., Li, C., … & Wen, X. (2022, February). An adaptive median filtering denoising algorithm for pepper and salt noised image. In Thirteenth International Conference on Graphics and Image Processing (ICGIP 2021) (Vol. 12083, pp. 372-378). SPIE.
CrossRef - Zhang, X. (2022). Two-step non-local means method for image denoising. Multidimensional Systems and Signal Processing, 33(2), 341-366.
CrossRef - Chang, H. H., Chu, W. C. (2009, March). Double bilateral filtering for image noise removal. In 2009 WRI world congress on computer science and information engineering (Vol. 6, pp. 451-455). IEEE.
CrossRef - Naidu, G. G., Ramesh, G. P. (2022, July). Mango Leaf Disease Detection Using Ultrasonic Sensor. In 2022 IEEE International Conference on Data Science and Information System (ICDSIS) (pp. 1-5). IEEE.
- Arya, S., Singh, R. (2019, September). A Comparative Study of CNN and AlexNet for Detection of Disease in Potato and Mango leaf. In 2019 International conference on issues and challenges in intelligent computing techniques (ICICT) (Vol. 1, pp. 1-6). IEEE.
CrossRef - Ahmed, S. I., Ibrahim, M., Nadim, M., Rahman, M. M., Shejunti, M. M., Jabid, T., Ali, M. S. (2023). MangoLeafBD: A comprehensive image dataset to classify diseased and healthy mango leaves. Data in Brief, 47, 108941.
CrossRef - Koundal, Deepika, Savita Gupta, and Sukhwinder Singh. “Computer aided thyroid nodule detection system using medical ultrasound images.” Biomedical Signal Processing and Control 40 (2018): 117-130.
CrossRef - Kaushal, Chetna, Shiveta Bhat, Deepika Koundal, and Anshu Singla. “Recent trends in computer assisted diagnosis (CAD) system for breast cancer diagnosis using histopathological images.” Irbm 40, no. 4 (2019): 211-227.
CrossRef - Shukla, Prashant Kumar, Jasminder Kaur Sandhu, Anamika Ahirwar, Deepika Ghai, Priti Maheshwary, and Piyush Kumar Shukla. “Multiobjective genetic algorithm and convolutional neural network based COVID-19 identification in chest X-ray images.” Mathematical Problems in Engineering 2021 (2021): 1-9.
CrossRef